Leveraging In-Silico Tools for Optimised Process Development
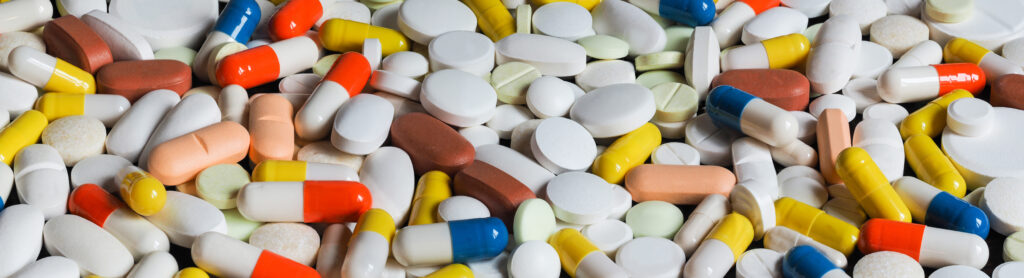
Presented by: Karsten Flügel, Principal Scientist, Digital Pharmaceutical Development at Merck Healthcare
Edited by: Cara Digby-Patel
Process simulation is a highly effective step in the process development stage of drug production. It allows for process optimisation in multiple ways and contexts, making it an invaluable tool during process development. Speaking at Oxford Global’s Formulation and Delivery 2022: In-Person UK event, Karsten Flügel, Principal Scientist, Digital Pharmaceutical Development at Merck Healthcare KGaA, explained the key benefits of utilising process simulation. Merck uses three modelling approaches: advanced process modelling (APM), the discrete element method (DEM), and computational fluid dynamics. Flügel focused on the first two, explaining their functioning and then offering case studies to reflect their utility.
What is Process Simulation?
Flügel described process simulation as “the model-based representation of technical processes and unit operations,” such as blending, granulation and coating. So long as the model is designed to mirror the reality of the unit operation at the degree of accuracy required for application, process simulation can vastly increase the understanding of how the process will run.
- Evaluating Closed System Drug-Transfer Devices
- Challenges in the Formulation of Biologics: Protein Aggregation, Safety, and Technology
- Oxford Global's R&D Key 40 Stories
Flügel laid out four main reasons that Merck applies process simulation models during process development. Firstly, it can allow for an improved scientific understanding of unit operations, providing knowledge beyond that which theory alone can offer. The process characterisation stages can also become more efficient, as process simulation often reduces the number of experiments required. Moreover, using models often results in the rationalisation of scale-up and tech transfer. Finally, process simulation allows for more efficient process development, which speeds up drug formulation.
Advanced Process Modelling
Advanced process modelling is the simplest of the three approaches. It utilises mathematical models based on chemical engineering's first-principles theory to produce equation systems which can calculate heat and mass transfer, compaction models, and population balance models.
One unit operation where Merck has used APM is for roller compaction to predict ribbon solid fraction. Flügel noted that models such as these would usually require some historical data to “train” the model. However, when using APM, the model could simulate ribbon solid fraction based solely on material characteristics which were measured independently, without having to perform any roller compaction experiments beforehand. This was reflected in the results from this model (see Figure 1), where a strong correlation between the simulated and the experimental ribbon solid fraction can be observed.
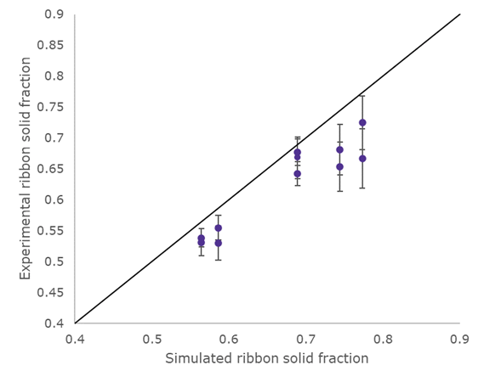
Advanced process modelling can also predict the LoD profile of fluid bed granulation. The model utilised historical data from small-scale lab experiments to accurately predict the LoD profile in large-scale experiments. Similarly to roller compaction, the primary benefit here is that APM can “predict process parameters which yield comparable LoD profiles during scale-up and ensure similar granule properties”, as is shown in Figure 2. Moreover, these predictions are largely accurate, meaning that a reduced number of experiments actually have to be performed.
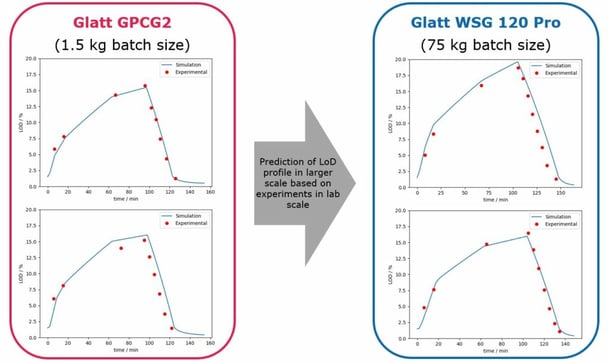
The Discrete Element Method
The discrete element method can describe and predict the behaviour and interaction of particles in three-dimensional space. After spawning particles, the software checks for any contact between them, and if there is, it calculates the resulting forces based on the degree of overlap of the particles. The forces are calculated based on Newton’s laws and then used to update the position of the particles for the next step when the cycle restarts. If run correctly, the discrete element method offers a highly accurate representation of the behaviour of particles moving inside a rotating coating drum.
Process simulation allows for process optimisation in multiple ways and contexts, making it an invaluable tool during process development.
Flügel gave two examples to highlight the efficacy of the DEM. One was a retrospective study where Merk used the DEM to perform a root cause analysis. The study was designed to establish why coating homogeneity in a larger coater, the Glatt GCS350, was worse than in a smaller one, the Glatt GCC150. Researchers modelled the two coaters with all conditions kept constant except for batch size; the GCS350 had a batch size of 160kg, whilst the GCC150’s batch size was 95kg.
Using the discrete element method, researchers established that the poor coating homogeneity in the Glatt GCS350 was likely the result of a “dead zone”, an area within the drum where mixing was markedly delayed (see Figure 3). The simulation results provided the team at Merck with useful knowledge regarding the coating capacities offered by different coaters which they could then apply in future projects.
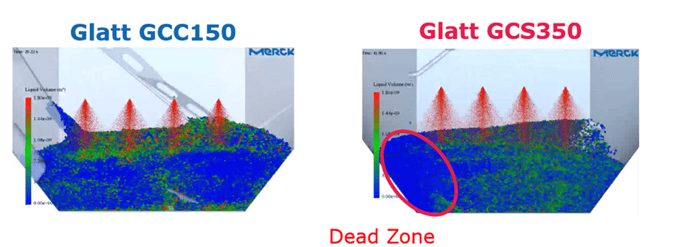
Flügel also noted that this type of simulation can be used to assess the mechanical stress inflicted on tablets. This allows researchers to determine whether the equipment used is suitable for the process development by optimising process settings to minimise mechanical stress.
The second case study investigated whether mixing behaviour changed if one component of the mixture’s particle size increased. The simulation was performed using different particle sizes, and quantitatively evaluated using the Lacey mixing index, a means of calculating a single value for the current state of mixing, with values ranging from 0 (a completely segregated system) to 1 (an ideally mixed system).
Using the discrete element method allowed the Lacey index value to be tracked throughout the mixing process and compare values to see if there were any differences in the mixing behaviour depending on particle size. Despite a shift in the curves (see Figure 4), researchers concluded that there was no observable difference.
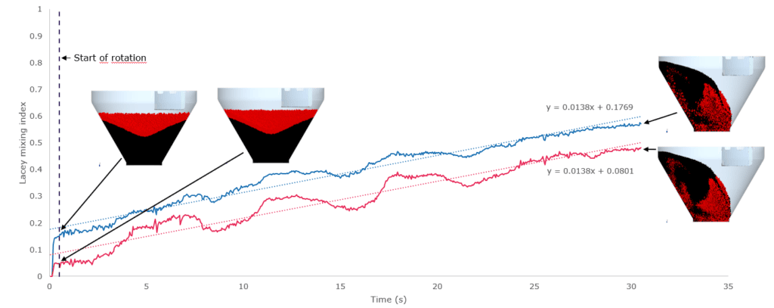
This conclusion was reached despite the two mixtures having different Lacey indices, because before rotation had begun there was already a difference in the values. Moreover, once rotation began, the difference between the two curves remained the same, and both had similar slopes too.
Conclusion
Flügel closed with a summary of the main benefits of using mathematical models during process development. These in-silico tools allow developers to rationalise the process development of drugs, for example, during scale-up and tech transfer. This increases the likelihood of “getting it right first time”. They also increase the scientific knowledge of processes, as understanding the underlying physical principles is pivotal for successful simulations. Finally, these models can supplement quality by design approaches through the assessment of the critical process parameters in-silico and defining paths such as proven and acceptable ranges.
Join leaders, experts, and researchers at Formulation & Delivery US: In-Person, connecting global pharma, biotech, and academia for high-level discussions on the latest innovations for biopharmaceutical development.