Using AI to Drive Drug Design: Maximising the Impact of Big Data with Computer Aided Drug Discovery
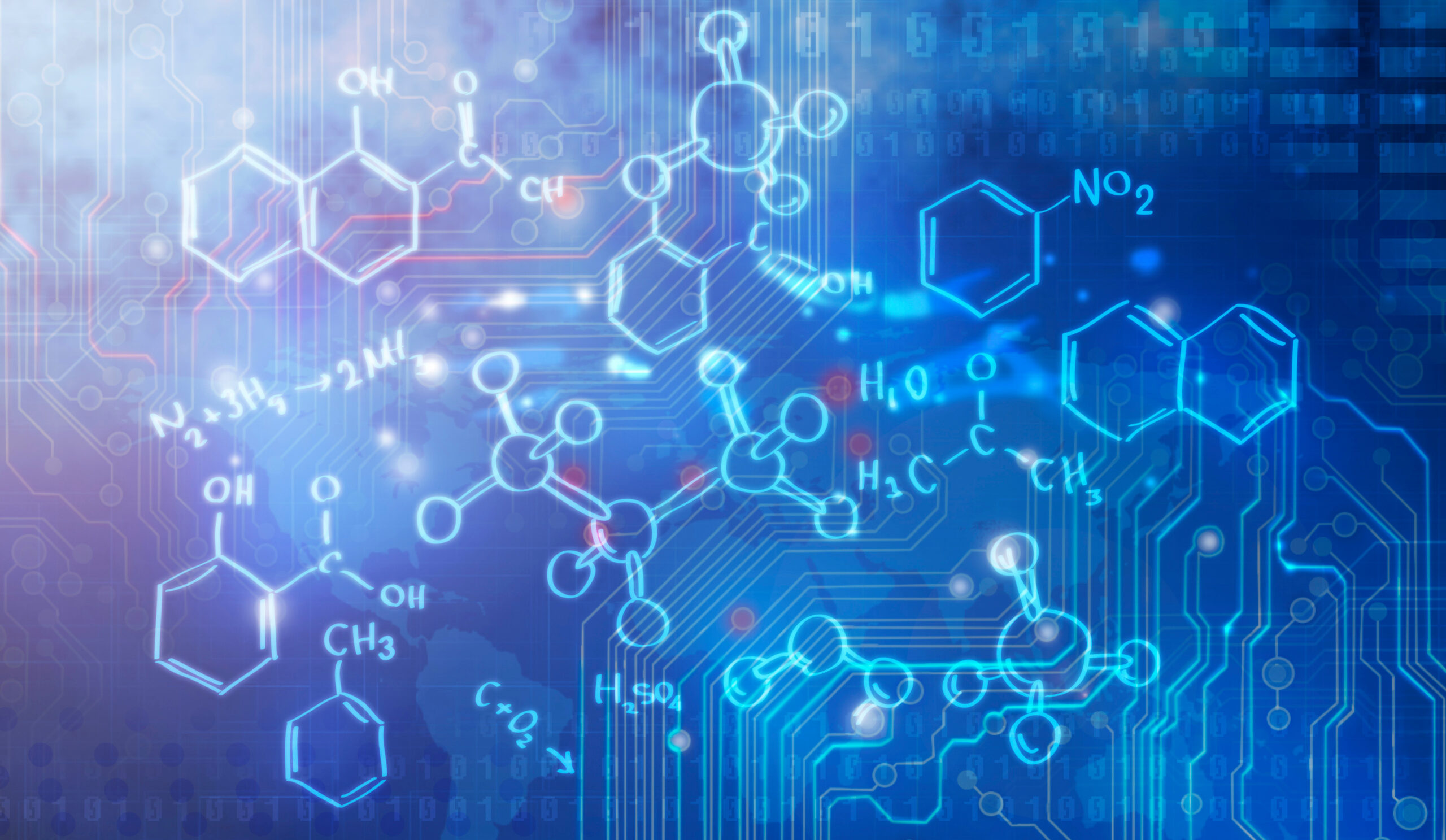
Edited by Tom Cohen
“What really interests me is how do we do the right science and ask the right questions, to put good drugs on the market,” said Richard Lewis, Director of Data Science and Computer Aided Drug Design at Novartis. Lewis presented his talk at Drug Discovery Europe 2022, titled Using AI to Drive Drug Design: Maximising the Impact of Big Data with CADD. Lewis’s presentation focussed on using AI to get the most out of the massive datasets available to bioinformaticians, all towards the goal of designing effective drugs.
Applying AI Methodologies to Lead Optimisation
According to Lewis, it has been estimated that the ‘universe’ of compounds contains approximately 1060 molecules that could be made. “Even if that figure is hopelessly exaggerated – let’s call it 1030 – the largest library that we can consider at the moment, is about 1015.” This means that sampling the largest library only accesses 1/1015 (one quadrillionth) of the molecular universe. “So, we have very low data availability,” said Lewis.
- AlphaFold: Momentary or Revolutionary?
- AlphaFold and Drug Design: Has AI Solved Biology’s ‘Grand Challenge?’
Furthermore, Lewis noted that this data is based on the molecules that have already been made, which means that the data is biased. On top of that, Lewis said that “the optimisation landscape that we are trying to design drugs in is discontinuous.” The factors for optimising a drug product could all be moving in different directions, making it hard to balance them all at once.
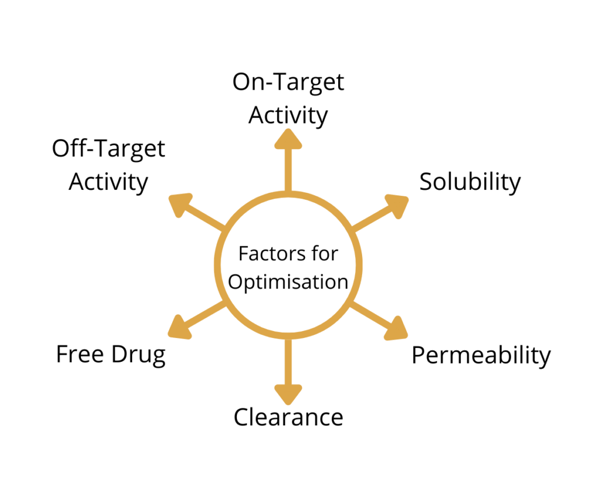
“So, we’ve been starting to use de novo methods,” explained Lewis. He added that these procedures were not new and have been around since the 90s. He said that over the last 30 years of using de novo methods, “the challenges are in getting the diversity and chemical feasibility of the generated molecules.”
Lewis described how the physical-chemical properties are often not considered during the computer aided drug discovery optimisation process – structures are generated due to some basic constraints. Furthermore, he said that “most methods are based on existing or common chemical building blocks that are easy for us to buy quickly – so how diverse is that really?”
Another issue that Lewis mentioned was that true de novo generated molecules were often “a bit exotic.” This was particularly apparent when using a program called SkelGen: Lewis recounted the generated molecules using that program having “lots of spirocycles, stereocentres, unusual heteroatom patterns. But they were de novo, you just never made them.” Lewis said that now, the “grammar” of databases like PubChem or ChEMBL is employed to constrain the generated molecules, making them more reasonable and less exotic.
In developing their ML workflow, Novartis teamed up with Microsoft Research: “the goal is to connect users through a nice UI and data analysis tool,” said Lewis. In doing this, Lewis explained that the end result would be a target property profile: “what do you want in your ideal molecule?”
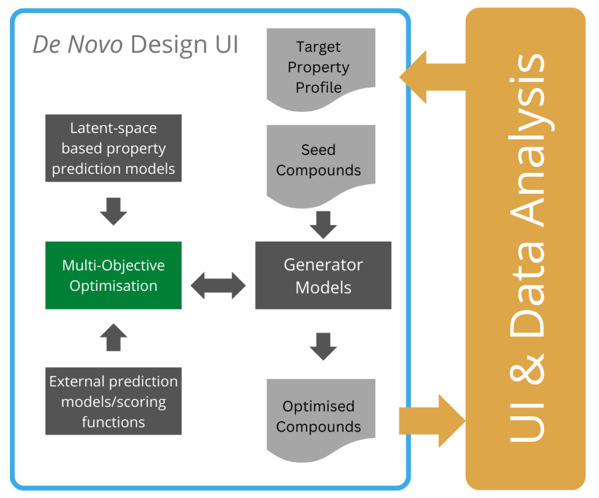
Their de novo method starts with a database of already-assayed seed compounds which are put through generator models.
Novartis’s multi-objective optimisation framework uses property prediction models in latent space and post-processing. The products of the program are ‘optimised compounds’ - or as Lewis called them “optimised suggestions” – “The idea that any program would come up with the finished drug is just fanciful. What the program does is suggest to the chemists ways to go and things to try.”
Generative Chemistry
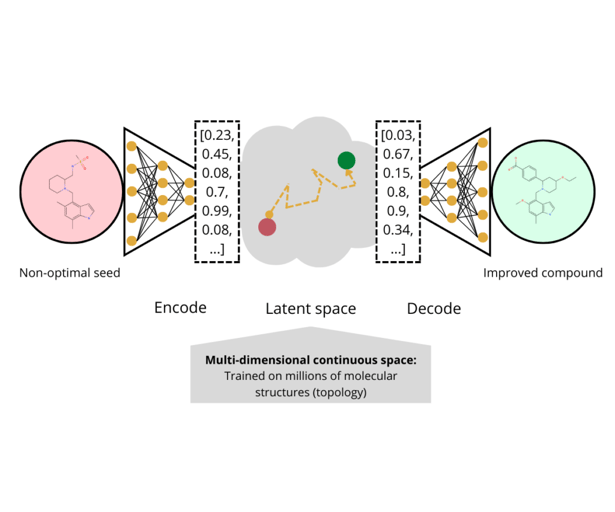
Lewis then took the audience through the principle and overall outline of generative chemistry. “You start with a non-optimal lead and then encode it in some way,” Lewis explained. Encoding the compound converts it into a mathematical vector, divorced from its molecular chemistry. The converted compounds then encode the latent space of the model, what Lewis calls the “grammar” of that space is based on databases such as ChEMBL or PubChem.
“You do this optimisation and throw into the space other predictive models for LogP, solubility, permeability, hERG, et cetera.” After the optimisation process, the output is another vector that needs to be decoded before it is shown to a chemist. The final output is the improved compound.
One question that Lewis brought up with regards to this generation method was whether or not it could produce an optimised compound that was better than what a medicinal chemist could come up with. To this, Lewis said that “if you’re analoging around the seed compound, the AI program probably won’t do any better than the chemist.” However, for exploring the area beyond the seed, the AI program is more likely to have more traction.
Data Needs Before Model Building: ‘Data Needs to Be Big and Diverse’
Lewis said that data needs had more or less remained the same since the inception of QSAR model building. “When you’re doing model building,” expanded Lewis, “what they say is that you need to spend at least 80% of the time preparing the data.” The definition of big data that Lewis gave was as follows:
‘The information assets characterised by such a high volume, velocity, and variety to require specific technology and analytical methods for its transformation into value’
Lewis added that this definition currently amounts to datasets with over 100,000 observations.
Furthermore, Lewis advised that for single assay data, often more established models are appropriate: “You don’t need AI, you can do Random Forest, Naïve Bayes, Gaussian processes, et cetera.” However, he added that it was key to bear in mind that the fewer the observations made, the more vital curation is.
“For most cases in chemistry, we do not have big data,” said Lewis, commenting on the landscape of big data available. “Even the MELLODDY project, aggregating data from seven or eight big pharma companies, still was not big enough to call big data – it wasn’t diverse enough.”
Conclusion
Lewis concluded his presentation by stating that “good chemical ideas are at the heart of drug discovery.” He said that compound generation advances this, and that it is viable to use a variety of methods to do so: “each method has its pros and cons, so they can be applied in different tasks.”
All the more critical are reliable models with rich annotation. “Understanding the data, being close to the data, being close to the people who generate the data is completely vital.” Lewis suggested that an idea pool for selection and prioritisation by the medicinal chemistry team would help in this. “So, working with the MedChem team is vital: discussing ideas, understanding needs, and adapting,” he concluded. “The goal is to generate high quality ideas.”
Join and network with over 200 industry leaders at Discovery US: In-Person, where we will address the latest advancements in target identification, validation and HIT optimisation.