AI-driven High Throughput Screening for Targeted Drug Discovery
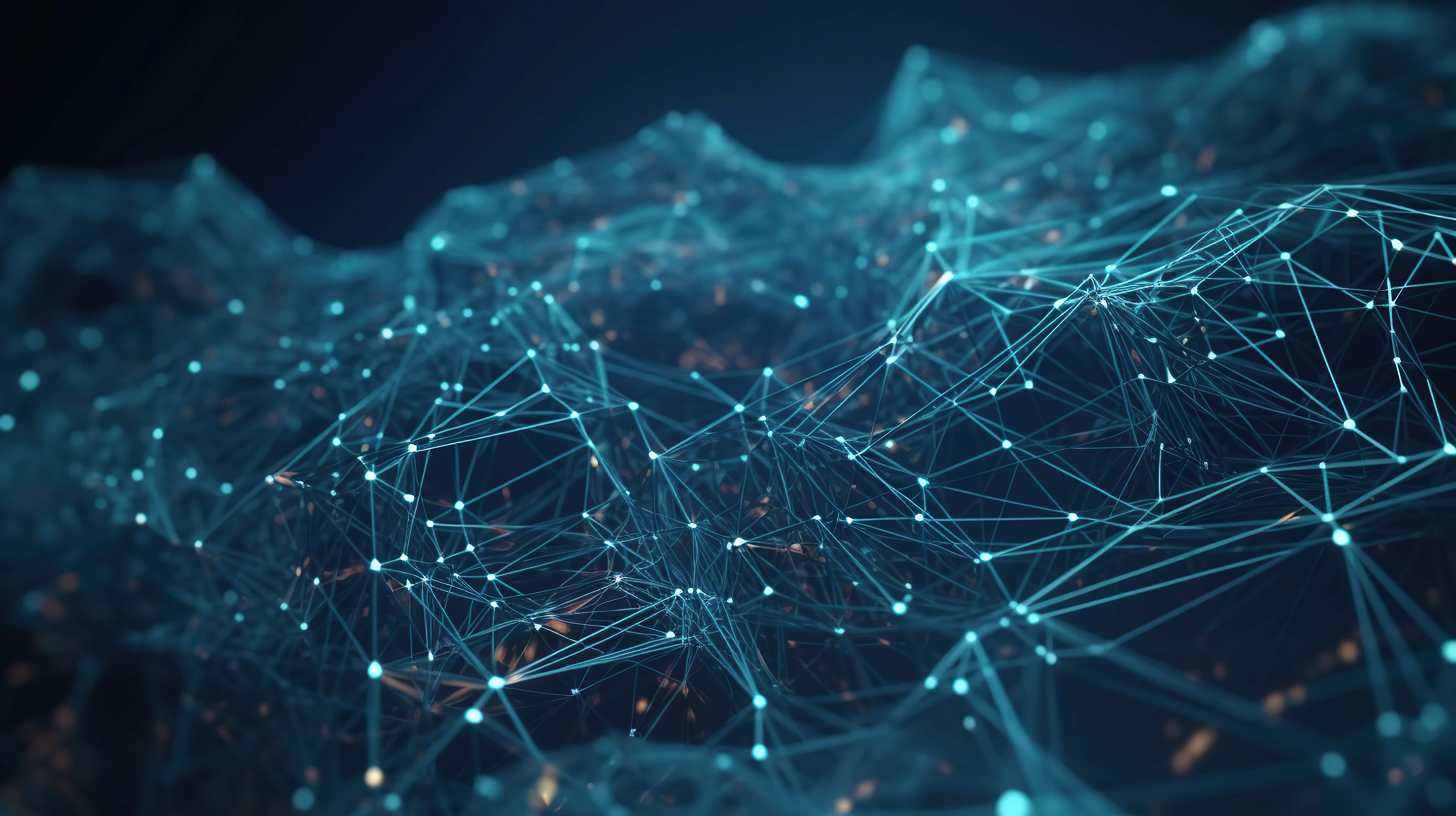
The need to develop new therapeutic drugs is an intricate, time-consuming, and costly process, traditionally reliant on high throughput screening (HTS) to evaluate vast libraries of compounds. HTS has been instrumental in identifying active compounds, antibodies, or genes that modulate a particular biomolecular pathway. However, the conventional HTS approach is fraught with challenges, including high costs, low success rates, and the need for extensive labor and resources.
The advent of Artificial Intelligence (AI) presents a groundbreaking solution to these challenges, offering new avenues for enhancing the efficiency and effectiveness of drug discovery. AI-driven HTS leverages machine learning (ML) algorithms and computational models to analyze and interpret complex biological data, significantly accelerating the drug discovery pipeline while reducing associated costs and errors.
Discovery by Oxford Global take a deep look at the role and impact of AI-driven technologies in revolutionizing HTS processes as they represent a pivotal shift towards more targeted, efficient, and personalized drug discovery approaches, promising to transform the pharmaceutical industry's landscape.
What is High Throughput Screening?
High throughput screening (HTS) is a methodological cornerstone in drug discovery, enabling the rapid evaluation of the biological or biochemical activity of a large number of compounds against selected targets. HTS involves the use of automated equipment to conduct various biochemical, genetic, or pharmacological tests on thousands to millions of samples in a relatively short time. This approach has historically expedited the identification of active compounds, antibodies, or genes that affect specific biological pathways.
However, traditional HTS processes are not without limitations. The sheer volume of compounds and the complexity of biological interactions often result in high false positive or negative rates. Conventional HTS methodologies can be prohibitively expensive and time-consuming, requiring substantial investment in reagents, equipment, and manpower. These challenges underscore the need for more sophisticated and efficient methods to enhance the drug discovery pipeline's effectiveness and throughput.
AI-driven HTS promises to address these challenges by integrating advanced computational techniques with traditional screening methods. By applying AI and ML algorithms, scientists can improve the accuracy and speed of data analysis, reducing the likelihood of false leads and enabling more precise targeting of biological pathways. This evolution in HTS methodology signifies a significant step forward in the drug discovery process, offering a more streamlined, cost-effective, and accurate approach to identifying promising therapeutic candidates.
How do AI and Machine Learning affect High Throughput Screening?
Artificial Intelligence (AI) encompasses a broad range of computational techniques designed to simulate human intelligence processes. Within the context of drug discovery, AI primarily refers to machine learning (ML) algorithms that learn from and make predictions or decisions based on data. ML, including subfields like deep learning and reinforcement learning, plays a crucial role in deciphering complex biological datasets and identifying patterns that may not be immediately apparent to human researchers.
Deep learning, a subset of ML, employs neural networks with multiple layers (hence 'deep') to model high-level abstractions in data. In HTS, deep learning algorithms can process and analyze vast amounts of chemical and biological data to predict compound behavior, enhancing the selection process for potential drug candidates.
Reinforcement learning, another ML approach, involves algorithms that learn to make decisions by performing certain actions and assessing the outcomes. In the realm of HTS, this can translate into improved assay design and optimization, where the system learns to modify experimental parameters to achieve the best possible screening outcomes.
These AI methodologies, powered by the increasing availability of biomedical data and advances in computational power, enable a more nuanced and sophisticated analysis of drug interactions and mechanisms. By leveraging AI and ML, researchers can streamline the HTS process, enhance the accuracy of screenings, and ultimately accelerate the identification of viable drug candidates.
How to integrate AI into High Throughput Screening?
The integration of AI into HTS processes signifies a transformative shift in drug discovery. AI-driven HTS utilizes sophisticated algorithms to enhance data processing, analysis, and interpretation, leading to more efficient and accurate screenings. This integration allows for the rapid identification of promising compounds from vast chemical libraries, significantly speeding up the drug discovery timeline.
One of the key advantages of AI-driven HTS is its ability to learn from and adapt to new data. Traditional HTS methods are static, relying on predetermined conditions and parameters. In contrast, AI algorithms can continuously update and refine their predictions based on new information, leading to a more dynamic and responsive screening process.
AI-driven HTS can also significantly reduce the rate of false positives and negatives, a common challenge in traditional screenings. By accurately predicting compound efficacy and toxicity, AI algorithms can filter out unsuitable candidates early in the screening process, focusing resources on more promising compounds.
Case studies in the pharmaceutical industry illustrate the successful application of AI-driven HTS. For example, companies have used AI to optimize the screening of kinase inhibitors, resulting in the identification of novel compounds with high specificity and potency. Such advancements demonstrate the potential of AI to revolutionize the drug discovery process, offering a faster, more cost-effective route to new therapies.
Challenges and Solutions in AI-driven HTS
Despite its potential, the integration of AI into HTS is not without challenges. One major hurdle is the quality and availability of data. AI algorithms require large, diverse, and accurately labeled datasets to learn effectively. In many cases, the proprietary nature of drug discovery data and concerns over intellectual property limit data sharing, hindering the development and training of robust AI models.
Another challenge is the interpretability of AI decisions. AI algorithms, particularly deep learning models, are often considered "black boxes" because their decision-making processes are not easily understood by humans. This lack of transparency can be problematic, especially when justifying drug candidate selections to regulatory bodies. We tackled The Black Box Effect and How Can AI and ML Provide Transparent Insights for Drug Discovery
Collaborative initiatives and data-sharing platforms are being developed to improve data accessibility and standardization. Additionally, researchers are working on 'explainable AI' techniques to enhance the transparency and interpretability of AI-driven decisions.
Future Perspectives and Ethical Considerations
The future of AI-driven HTS in drug discovery appears promising, with potential to significantly accelerate the development of new medications and therapies. As AI technologies evolve, they are expected to become more integrated into every stage of the drug discovery process, from initial screening to clinical trials.
However, the rise of AI in drug discovery also raises ethical and regulatory considerations. Issues such as data privacy, consent, and the potential for algorithmic bias must be addressed to ensure that AI-driven processes remain transparent, fair, and beneficial to all.
In conclusion, the integration of AI into HTS is transforming the landscape of drug discovery. By enhancing the efficiency, accuracy, and speed of screenings, AI-driven approaches are paving the way for more rapid and cost-effective development of new therapies. Despite facing challenges, the continued evolution and integration of AI technologies hold immense promise for the future of pharmaceutical research and personalized medicine.
Conclusion
AI-driven high throughput screening represents a significant advance in the field of drug discovery, offering the potential to overcome traditional HTS limitations and streamline the identification of novel therapeutic agents. By leveraging AI and ML algorithms, researchers can enhance the efficiency, accuracy, and cost-effectiveness of drug screenings. While challenges remain, particularly in terms of data quality, interpretability, and ethical considerations, the ongoing evolution of AI technologies promises to further revolutionize the drug discovery process. As these technologies continue to mature and integrate into research workflows, they hold the potential to significantly accelerate the development of effective and personalized medical treatments.
Get your weekly dose of industry news here and keep up to date with the latest ‘Industry Spotlight’ posts. For other Discovery content, please visit the Discovery Content Portal.