The Highs and Lows of Applying AI to Drug Design
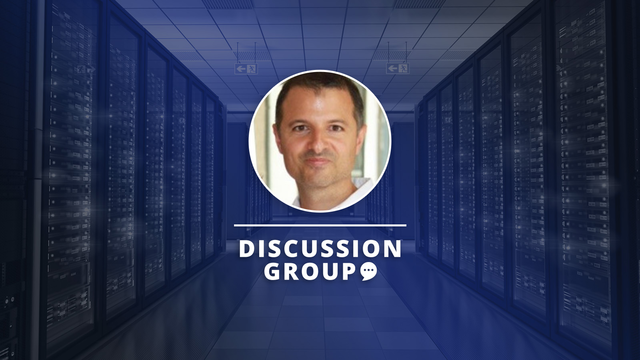
Robert Soliva, Head of Drug Discovery at Oryzon Genomics, hosted our September discussion group on Applying AI to Drug Design. Soliva began the discussion by recounting his own professional experience with using AI/ML-based methods for drug design. “In my experience,” said Soliva, “AI is useful but not as useful as it is usually presented.” He added that – in his opinion – AI was at the ‘peak of inflated expectations’ on the Gartner hype cycle. Although, after the peak there is a trough, the next phase is productivity. Soliva took us through the highs and lows of the development trajectory of AI applied to drug design.
- Merck KGaA: Accelerating Drug Discovery with Automation and Artificial Intelligence
- Artificial Intelligence for Drug Design and Discovery
‘Not-So-Shiny Examples of AI/ML’
Soliva commented on the landscape of AI/ML methods’ near misses and before-its-time disappointments. In 2016, IBM promised to adapt their acclaimed Jeopardy!-playing AI, ‘Watson’ for target finding with ‘IBM Watson for Drug Discovery’. This was a project that Soliva was a part of when he worked at Nostrum Biodiscovery, the spin-off of the Barcelona Supercomputing Center he participated in founding.
Soliva said that his team were approached by IBM in early 2017 and were in communication with them while they tested the platform. “IBM said that this technology could work wonders and discover protein-protein interactions. And that it would lead us to find new mechanisms of action for some of our programs,” Soliva explained.
“The truth is that it was almost useless,” he added. Unfortunately, it came as no surprise when in 2019, IBM shut the Watson for Drug Discovery project down. Soliva said that after extensive testing “we didn’t find a single example of it giving us some insight or generating an interesting hypothesis that could open up new paths in our discovery projects.”
Furthermore, ML methods have been used to produce scoring functions used in virtual screening and docking simulations. “These were presented as being more reliable as the usual, prototypical scoring functions,” said Soliva. Again, unfortunately, Soliva explained that the ML-based methods had not performed as brilliantly as had been predicted: “They did not outperform the regular scoring functions that we had already been using.” Results from the ML functions were best when used on the systems that were used to train the model. But, as Soliva put it, “when you moved out of the training system, our view is that everything fell apart.”
- AlphaFold: Momentary or Revolutionary?
- AlphaFold and Drug Design: Has AI Solved Biology’s ‘Grand Challenge?’
“We have recently been spending some time with AlphaFold structures,” added Soliva, “using them for virtual screening.” In his experience, and the experience of his colleagues at Nostrum, researchers ought to be careful about AlphaFold in terms of managing structures: “because most of them have collapsed binding sites.” Because of this, Soliva reckoned that their performance was “suboptimal” if not managed carefully.
Working Use-Cases of AI/ML
Despite these disappointments, there are indeed areas where AI and ML are proving to be helpful. Soliva gave the example of a project that he worked on at Nostrum: “we wanted to reliably predict how agonists bind to GPCR.” The group’s first test case concerned the µ-opioid receptor along with a morphine-like compound and a peptide, both acting as agonist ligands. “That receptor has been co-crystalised and is available in the PDB (protein data bank),” explained Soliva.
Soliva’s team used a Monte Carlo method-based simulation platform called PELE MC, which could “very quickly sample” ligand-receptor interaction and generate a series of poses. The poses that PELE MC generates are then ranked based on their force-field energies plus scoring functions. Although this method gets the team part of the way there, Soliva commented that “you cannot tell which one is the actual binding mode as opposed to a series of artifacts when relying purely on scoring functions.”
To get closer to verifying which pose is the binding mode, the team uses a molecular dynamics simulation to extract what Soliva called a “fingerprint” – this summarises all the intra-molecular and inter-molecular residue contacts in the transmembrane domain of the GPCR. “It’s like a fingerprint of activation when some agonists are bound,” added Soliva. The team then use this fingerprint to rank all of the binding modes that are generated by PELE for each of the agonists. “So, this did help us determine which poses were actually binding modes and not a series of artifacts,” concluded Soliva.
AI/ML Applications at Oryzon Genomics
At Oryzon and thanks to a continued collaboration with Nostrum Biodiscovery, Soliva’s team uses autoencoders to developa novel series of de novo binders for novel protein-protein contact. Oryzon are interested in disrupting protein-protein interactions involved in deleterious gene expression programs, all while not knowing what could bind there, “all that we know is that the binding site is druggable,” added Soliva. “We didn’t know where to start.”
So, the team took a database with many million molecules and rank ordered them based on different scoring approaches. “Then we trained our autoencoder to generate the same molecules and introduced variations – new chemistry which is then re-docked and then scored again,” explained Soliva. The Oryzon team then ran this process as a cycle a series of times.
That workflow, Soliva said, allowed them to find some interesting solutions de novo. “The new chemistries have a series of desirable parameters,” he added, “synthetic accessibility, drug likeness, and so on.” From his point of view, AI-ML is definitely a powerful tool if applied carefully. However, overselling the technology might lead to too high expectations – “at the end of the day, our main limitation is still lack of knowledge on the biology of disease”.
After taking us through this timeline of AI/ML applications, Soliva opened the flaw to discussion from the rest of the session’s participants. To be a part of our next interesting discussion, please see our membership page for access to all of our monthly discovery discussion group sessions.
Our Automation in Discovery Symposium will host over 300 key leaders and influencers from global pharmaceutical organisations and leading academic research institutions presenting on the latest developments and critical approaches and technologies in automation to reduce attrition and improve productivity in drug discovery.