Single Cell Transcriptomics: Computational Analysis and Data Normalisation
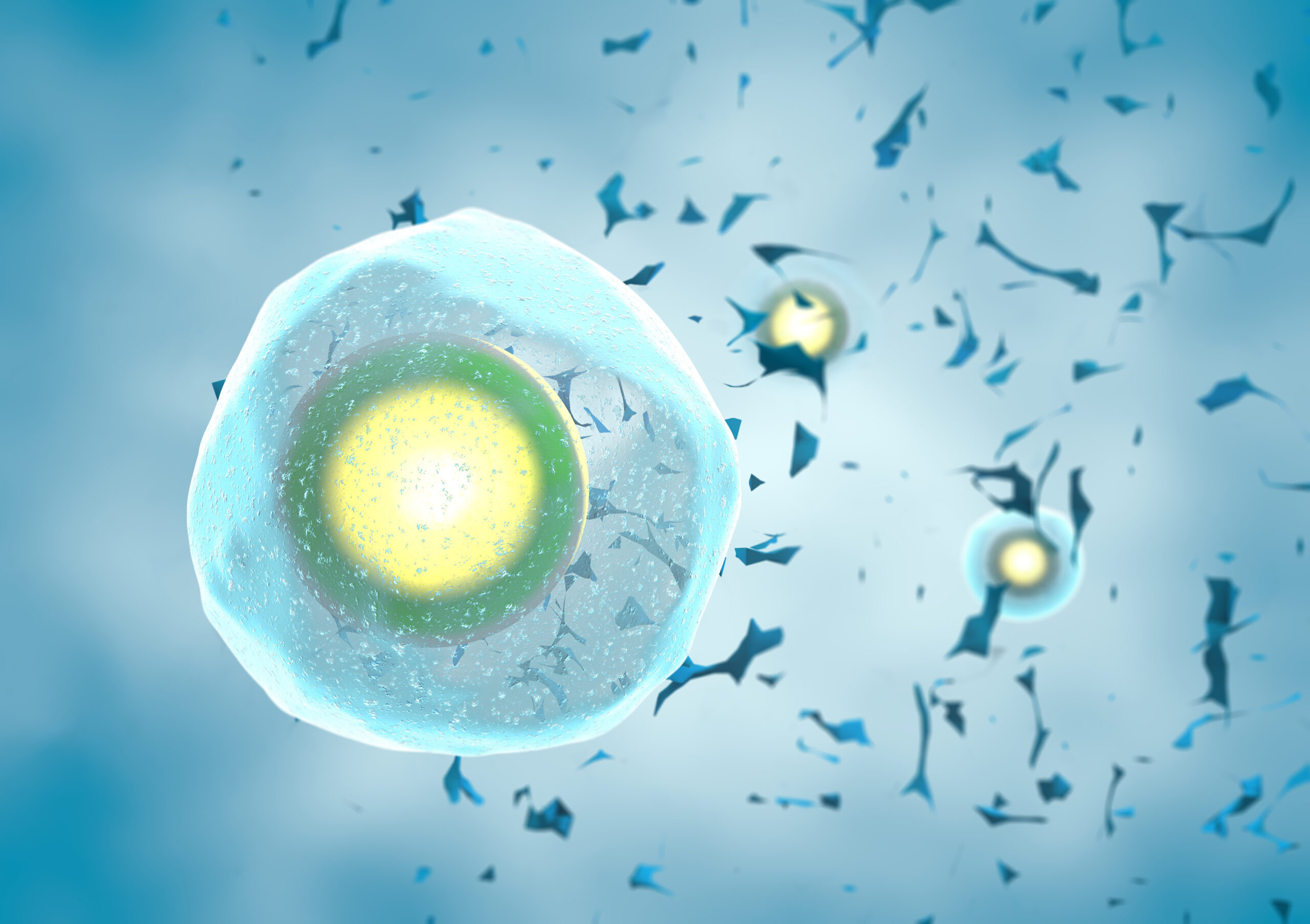
Single-cell analysis is the study of genomics, transcriptomics, proteomics, metabolomics and cellular interactions at the single-cell level. Due to the heterogeneity seen in both eukaryotic and prokaryotic cell populations, analysing individual cells opens up possibilities to understand previously unobservable mechanisms. As a result, the field has exploded in popularity in recent years and continues to expand and garner interest and investment.
Eric Chow explains single-cell analysis using a ‘fruit smoothie’ analogy; “let’s say that you’re working with a blood sample, and you want to take a look at the expression profile of within that blood sample. Blood is a complex mixture of many different cell types. You have B cells, T cells, macrophages, and neutrophils, similar to a smoothie, where you have lots of different types of fruit. And let’s say you’re really interested in the characteristics of, say, a raspberry or an orange. But instead of analysing that raspberry or orange, you mix it up with pineapple or bananas and strawberries, blend them all together, and then that’s what you’re tasting. It’s going to be very difficult to figure out what tastes are coming from that raspberry. And so, the same thing when you’re trying to analyse data from a single cell versus a bulk sample. So, it’s really advantageous if you can get data specifically from individual cells, as opposed to making a bulk measurement. And one really cool thing about single-cell sequencing is that with the data, you can identify lots of different cell types.”
Origins of Single Cell Transcriptomics
The origins of single-cell transcriptomics can be traced back to early methods such as single-cell qPCR in the early 1990s. While the exact date is still a matter of debate, many cite Tang et al.’s (2009) research into rodent germ cells as the first example of single-cell transcriptomics. Since then, several other single-cell transcriptomics protocols have been created, including tag sequencing methods such as STRT-seq, CEL-seq, MARS-seq and full-length protocols such as SMART-seq or SMART-seq2.
These protocols differed in their amplification technology and transcript coverage, as well as in the extent of robotisation of liquid handling in plates. This was followed by development of nanodroplets, picowell technologies and in situ barcoding which have made it possible to sequence tens of thousands of cells in parallel. In recent years multi-modal single-cell methodologies that measure and integrate genomics readouts from different molecules (RNA, DNA and protein), enabled dissection of the complex regulatory and cell-cell communication networks that drive cell identity to a greater degree than ever before.
What’s Next: Modern Single Cell Transcriptomics
Though SC-RNA-seq has deepened our understanding of cellular heterogeneity and molecular activity, it is impeded by several technical and computational challenges.
Data Normalisation
A key part of analysing and interpreting RNA single-cell RNA-seq data is normalising the data. Several methods for data normalisation of single-cell data have been created, some of which rely on spike-in genes, molecules added in known quantities to serve as a basis for a normalisation model. Depending on available information and the type of data, some methods may express certain advantages over others. Researchers from Imperial College London have proposed a Bayesian method, arguing that the basic methods that are commonly used do not work when you need to impute data with missing values.
Computational Analysis
Despite their utility, traditional expression experiments are limited to providing measurements that are averaged over thousands of cells, which can mask or even misrepresent signals of interest. Fortunately, recent technological advances now allow us to obtain transcriptome-wide data from individual cells. This development is not simply one more step toward better expression profiling, but rather a major advance that will enable fundamental insights into biology.
While the data obtained from single-cell RNA-sequencing (scRNA-seq) are often structurally identical to those from a bulk expression, the relative paucity of starting material and increased resolution give rise to distinct features in scRNA-seq data, including an abundance of zeros (both biological and technical), increased variability, and complex expression distributions. These features, in turn, pose both opportunities and challenges for which novel statistical and computational methods are required.
Conclusion
The surge of success in single-cell technologies has developed through the combination of academic research together with commercial initiatives to make robust, scalable and affordable technologies. Underpinning this has been the continual development of computational methods for data processing, analysis and integration.
Bacher, R., Kendziorski, C. Design and computational analysis of single-cell RNA-sequencing experiments. Genome Biol 17, 63 (2016). https://doi.org/10.1186/s13059-016-0927-y