Untargeted Metabolomics Data Analysis Reinforced by AI/ML Techniques
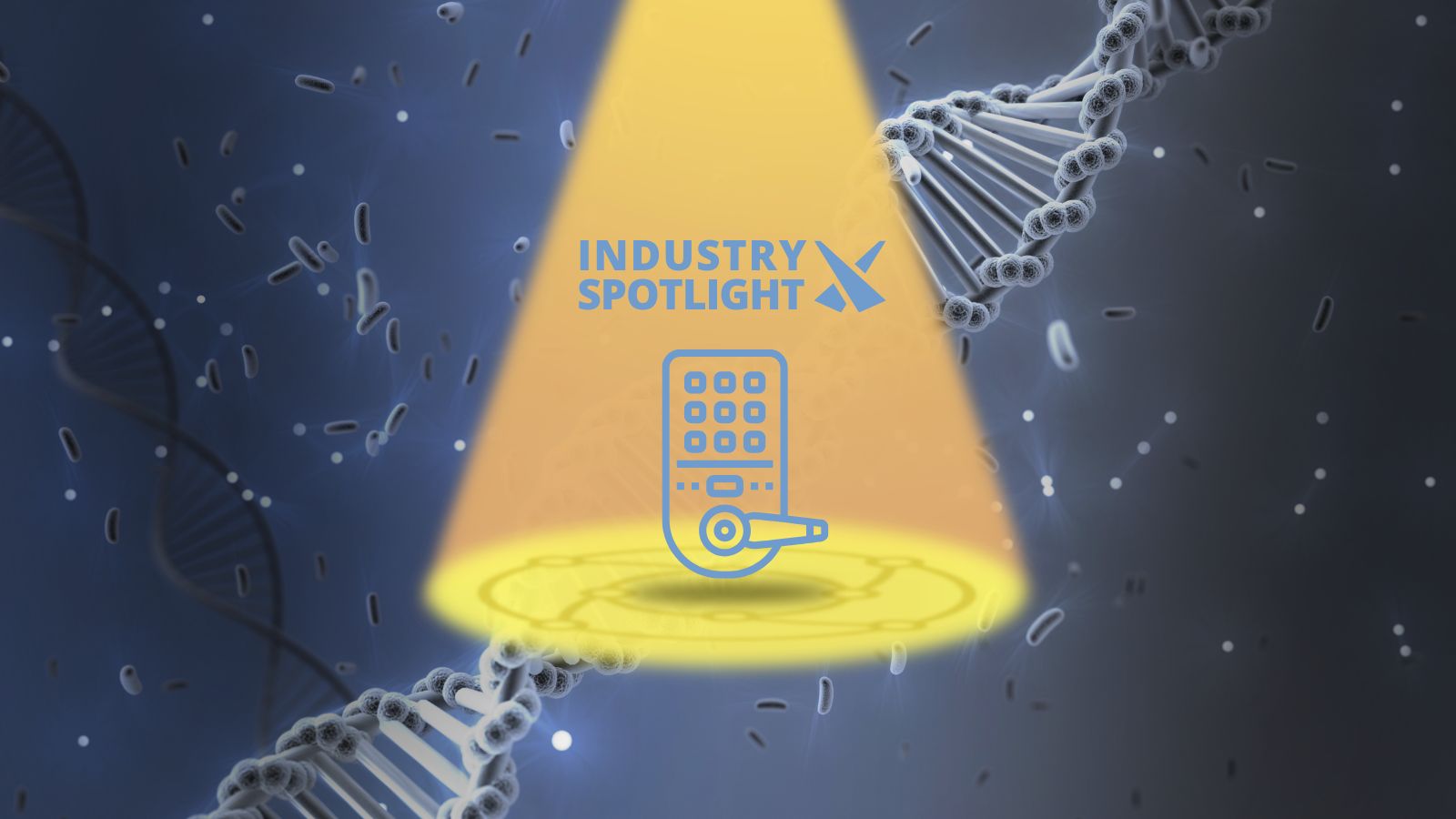
Untargeted metabolomics data analysis provides a robust picture of patient disease states, but interpreting the volume of information generated is challenging.
Fortunately, some of the applications of artificial intelligence (AI) and machine learning (ML) to the emerging field of high-resolution mass spectrometry are facilitating the easier processing and understanding of data.
Untargeted metabolomics typically involves comparing the metabolome of control and test groups to identify differences between their metabolite profiles.
The approach can be used for the quantification of small molecules in a given sample, with applications including mitochondrial biology.
- Listen: Ahmet Coskun talks about the future of spatial omics
- High-throughput cloning: could possibility become reality?
- New machine learning algorithm proposed for predicting CRISPR editing efficiency
Given the variety of chemical classes and physical properties that characterise metabolites and the dynamical range of metabolite concentrations over large orders of magnitude, a wide range of analytical techniques are required for metabolomics research.
The entire number of compounds analysed in untargeted metabolomics is extensive, as entire datasets — including thousands of metabolic signals — need to be processed.
Raw datasets in metabolomics are inherently complex because of the multiple linear and non-linear interactions among the metabolites.
This is where AI methods can assist in dataset analysis and data interpretation, through the quantification of untargeted metabolomics datasets.
How Do AI/ML Methods Assist in Untargeted Data Analysis?
AI and ML algorithms can assist with data alignment, as well as feature selection to pinpoint important exposures, metabolites, and biomarkers.
One approach to untargeted metabolomics involves acquiring data through imaging techniques such as mass spectrometry.
Once the raw data has been acquired, AI/ML can be incorporated into a number of subsequent steps in the metabolomics workflow.
Data from tandem mass spectrometry may be pre- or post-processed using a variety of algorithms to transform the large amount of raw spectral data into a much smaller, statistically manageable set of peaks and features.
Software processes here include selecting peaks and aligning them across samples: when data are adjusted to remove unwanted technical variation, feature selection takes place, typically through statistical approaches.
Additionally, ML may be used to focus on small molecules associated with health outcomes or exposure, providing an indication of patient disease states interpreted via metabolite profiles.
One example of the applications of AI in untargeted metabolomic data analysis is the pre-processing of raw data workflows, which is crucial for accurate translation of 3D data obtained through liquid chromatography mass spectrometry into a 2D-aligned peak table.
Even though data pre-processing can be easily performed using automated software, it is challenging to precisely, accurately, and robustly synthesise the data across the full range of metabolite features.
Examples of algorithms designed for this kind of pre-processing include open-source XCMS, MZmine3, MS-Dial, and MetAkign, as well as several types of proprietary software.
It is important to determine whether exposure data — and not just endogenous metabolites — are retained in the analysis, especially after data processing.
AI and machine learning are also useful for feature selection, as well as analysing the complex untargeted data in biological samples.
Get your weekly dose of industry news and announcements here, or head over to our Omics portal to catch up with the latest advances in spatial analysis and next-gen sequencing.