The Applications of AI Prediction in Spatial Imaging Approaches
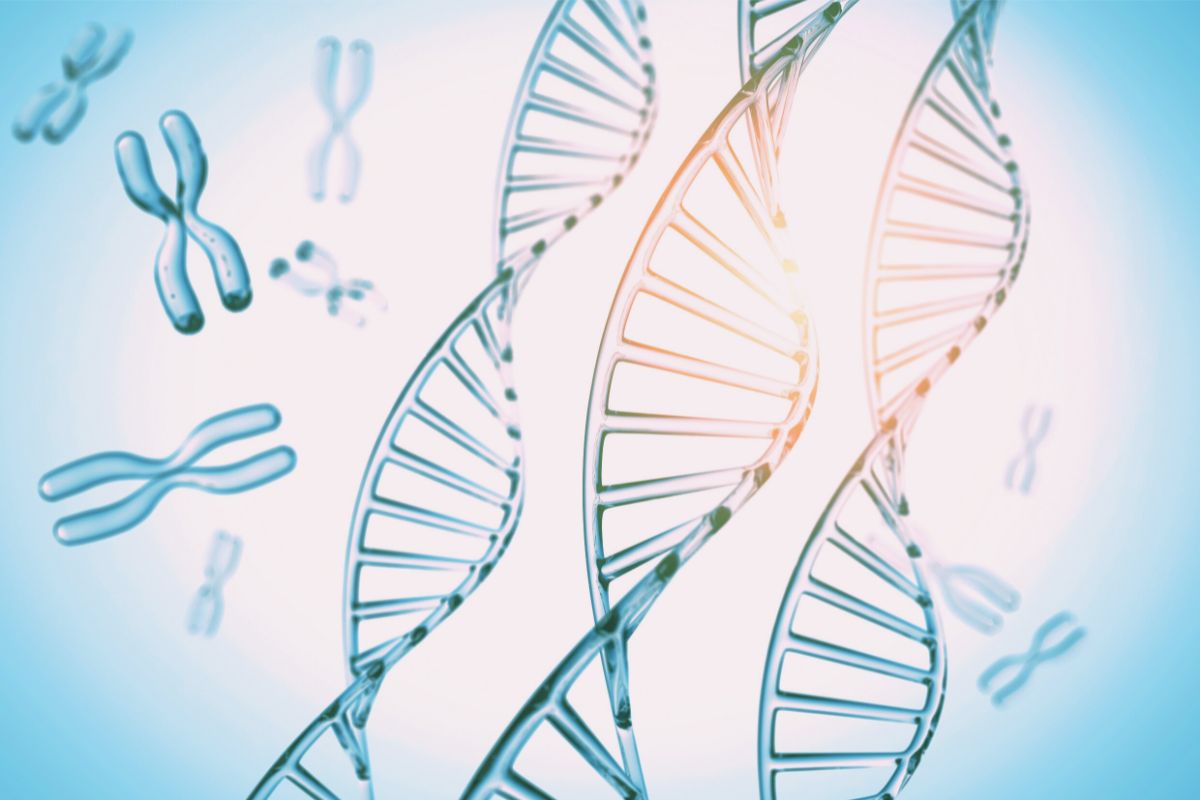
The applications of spatial imaging approaches in healthcare and pathology continue to grow as the precision and accuracy of the techniques used keeps improving. From the investigation of genome architecture to the complete 3D reconstruction of cell state, imaging approaches can be used to interrogate a vast array of patient information. However, as the techniques used grow in their complexity, so too do the datasets and information they generate. Fortunately, AI prediction and machine learning approaches are up to the task of interpreting imaging data. Here, we explore two presentations from Oxford Global’s NextGen Omics US 2023 event which look at some of the ways in which AI prediction is helping to reinforce the interpretation and interpolation of data.
Interpreting 3D Genome Folding
One area where AI prediction is proving useful in interpreting behaviour and interaction is genome organisation in 3D. Siyuan Wang, Assistant Professor of Genetics and Cell Biology at Yale School of Medicine, explained how the organisation and folding of the human genome in three dimensions dictates many essential genomic functions. “We think this is the new frontier – or the new ‘dark matter’ of genome research,” said Wang, “but this is actually a long-lasting question that has been explored for years.”
Previous sequencing-based approaches to study the 3D genome often required a population averaging of many copies of the genome, Wang explained, and many imaging-based techniques in this field provided chromatin ‘blobs’ rather than realistic 3D folding patterns. “Our understanding of 3D genome organisation is largely ‘blob-ology’ – how do we get the real 3D folding organisation of DNA in this space?” The solution is chromatin tracing, a approach to sequentially image points associated with genomic regions along single cell chromatins at nanometre-scale precision.
Wang continued by explaining that this project grew from a desire to investigate how 3D genome architectures differ between cancer cells and regular, healthy cells. The approach taken was to follow an adenoma – a benign, non-cancerous tumour – through to late-stage cancer cells. “We’ve collaborated to apply our genome-wide chromatin-tracing technique to a MADM (Mosaic Analysis of Double Markers) mouse lung cancer model,” said Wang. “This is a mouse model of LUAD, or lung adenocarcinoma – the number one cause of cancer deaths both worldwide and also in the United States.”
The MADM model enables lineage tracing through the fluorescent staining of cells, allowing for the identification of cell clusters. Wang described the chromatin tracing technique as being comparable with multi-model imaging, with the approach combining genome imaging and protein markers.
Using Supervised Machine Learning to Interpret Single Cell Folding
A key aspect of interpreting the 3D genome is understanding its structure. Obtaining precise information around the behaviour of the 3D genome is one thing; interpreting it is an equally important discipline that requires a consistent approach and a high degree of accuracy. Wang explained that conceptions around the folding behaviour of the 3D genome meant some researchers feel it is difficult to encode precise information such as how the associated single cell folding should look. The solution? Supervised machine learning.
“Just from using the single cell 3D genome configuration, we can predict the cancer state with about 92% accuracy,” said Wang of the approach. “This opened up the possibility that it could be used for diagnosis, prognosis, and to predict retreatment responses in cancer and other diseases.” In future studies, machine learning may help to derive functional and mechanistic insights for cancer from new kinds of high-dimensional data. This can be achieved through the identification of candidate cancer progression driver genes from the 3D genome.
3D genome conformations of single cells can encode and distinguish cancer states, such as the single-cell 3D genome, for better precision medicine. Single cell 3D genome mapping can provide functional and mechanistic insights into cancer progression, such as novel cancer driver genes and regulators of 3D cancer genome reorganisation.
Augmentation of Spatial Imaging with AI Prediction
Another major area of focus in the field of spatial imaging at present is the way in which imaging approaches could be augmented by AI and computer-aided quantification. Yu-Hua Lo is Professor of Electrical and Computer Engineering at the University of California, and has devoted much of his time to improving outcomes associated with image processing. “We know that imaging is a big part of spatial biology, and we do a lot of high-resolution imaging,” said Lo of his work. “On the other hand, when we want to actually isolate the cells to do the single-cell analysis, we do something like a high-throughput imaging analysis.”
- Artificial Intelligence in Mammography Could Double Breast Cancer Screening Efficiency
- Genome Sequencing Approaches: Choosing Between Long-Read and Short-Read Sequencing
- Untargeted Metabolomics Data Analysis Reinforced by AI/ML Techniques
His laboratory’s approach has been to combine both, although this results in a “tremendous amount” of data – typically a terabyte per composite image. To help in interpreting this information correctly, Lo has looked to machine learning to assist in image processing for understanding the interior of the cell. In collaboration with NanoCellect, Lo and his team have produced a tabletop cell sorter, which enables the analysis of intracellular information.
“We have a microfluidic cartridge, and this cartridge has a microfluidic device,” Lo explained. The device captures information from the cells at a speed of 20,000 frames per second. To achieve this, it uses an optic deflector: the waveform signal from the cell is scattered and collected by a dedicated flow tube. As a subsequent transformation, the waveform is then converted into an image.
Constructing a 3D Cellular Tomography
Providing an example of the applications of this approach, Lo explained how the device could be used to study how a glucocorticoid receptor is translocated with and without drug influence. “Without the drug this is un-translocated,” said Lo, “so the glucocorticoid receptor is basically distributed over the entire cell body and reaching the membrane. With drugs, the protein is actually localised away from the cell membrane.”
The approach also allowed for the staining of organoids such as mitochondria, enabling researchers to see mitochondria distributions and study the T cell receptor (TCR). In tandem with single-cell genomic analysis, high-throughput 3D cell imaging may transform both cell classification and cell discovery, providing significant amounts of new knowledge for cell types. A strength of the approach is that it also offers a 2D image as a cell projection, allowing Lo’s team to see the tomography of the cell. Lo described this as the difference between taking a CT scan as opposed to an X-ray.
A 3D scanner was used to produce high-fidelity 3D images, employing a cylindrical lens to create a structured ‘sheet of light’ that could properly capture the different aspects of the cell. To accommodate this approach, the apparatus must allow for a con-focal microscope despite the cell travelling at great speed. “As the cell travels through each pinhole, the scanning light will scan one cross-section of the cell,” said Lo, “and across each pinhole light will scatter through different sections.” Data from all sections can be collated to yield a 3D tomography.
Using AI Prediction to Extrapolate Imaging
The use of an AI inference engine eliminates the need for real-time image feature extraction, with sorting decisions made based on raw images. The approach is not constrained by the number of images which could potentially be implemented in real-time, with users able to conduct image analysis offline and draw the gating criteria as usual. The AI inference engine interprets human gating and translates this into machine intelligence, which is used to inform decisions for cell sorting.
Lo explained that any new project should consider how 3D imaging data should be sorted and interpreted. “After training, we don’t have to translate all those 30-plus image features,” he said. “AI gives us predictions based on trending, with a reset at the confidence level.” He added that the extent of human interaction with the data and information here was capped at setting the confidence level in percentage. “By doing that, we make a channel to transmit human knowledge to artificial intelligence systems.” These decisions are made based on 3D tomography, with AI able to make decisions within two milliseconds. “If we improve the architecture, we can do it in half a millisecond,” Lo added.
One additional consideration with this use case is the use of supervised or unsupervised training. “So far, we’ve used a lot of supervised training to help with discovery,” said Lo. “This is costly and sometimes not even realistic.” An alternative approach is to use either unsupervised learning or semi-supervised learning, which works based on a Deep Convolutional Autoencoder-Based Clustering (DCAEC) model. The model automatically ‘finds’ and predicts input images based on latent representations, allowing models to predict incidence based on recorded or observed properties. As one example of this application, the model can predict leukaemia to 99.9% accuracy. AI prediction and computer-assisted approaches to imaging will be increasingly important as the quality and volume of data generated continues to grow.
Want to keep up with the latest developments in spatial transcriptomics and imaging? Sign up to our Omics newsletter to receive a monthly summary of industry trends and new advances in the field straight to your inbox. If you’d like to know more about our upcoming NextGen Omics UK conference, visit our event website to download an agenda and register your interest.