Data Integration in Genomic Analysis: Computational Methods for the Integration of Spatial Metabolomics
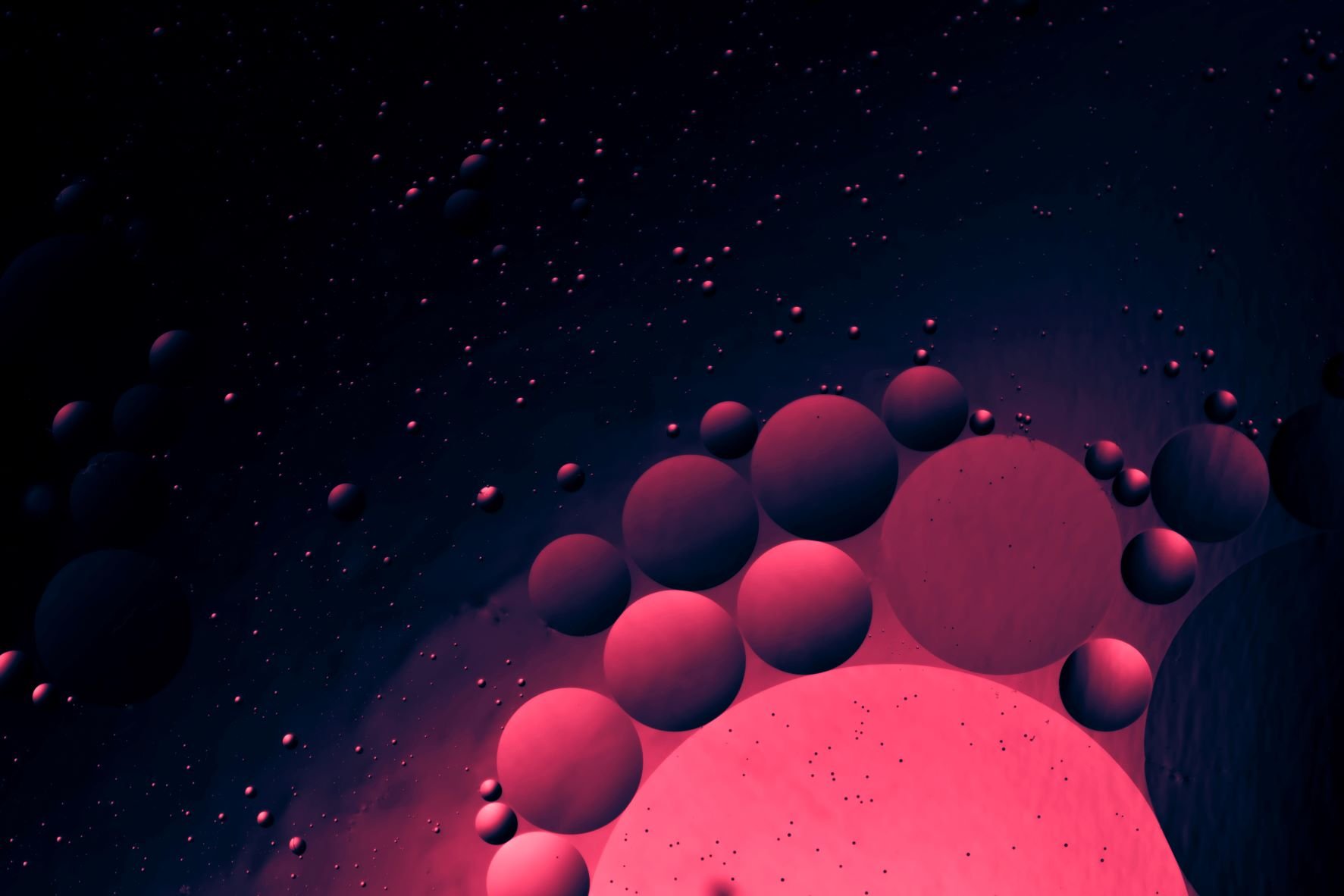
Presented by: Alex Dexter, Senior Research Scientist at the National Physical Laboratory (& National Centre of Excellence in Mass Spectrometry Imaging)
Edited by: Ben Norris
Multi-omics imaging techniques can be applied together to develop a complete understanding of genetic diseases and the efficacy of treatments. By integrating mass spectrometry imaging (MSI) data with non-imaging mass spectrometry (MS) data, and non-mass spectrometry imaging data, a more complete comprehension can be reached to return a more concrete analysis. As Alex Dexter explained to Oxford Global’s Omics Spatial Biology UK event in April 2022, the aim is to incorporate spatial metabolomics with other modalities.
“You acquire a mass spectrum at discrete locations and then you can build up, either by looking at a single peak or as a format spectrum which you can scroll through to interrogate your data,” said Dexter. By mapping out and conflating data in this way, Dexter and his team at the National Physical Laboratory can improve their search for potential biomarkers or other units of data. The applications of MSI include the visualisation of spatial metabolomic data and the mining of information.
“Why data integration?”, Dexter asked. “One of the things you might want to do is combine multiple modalities of information together.” He gave the example of combining morphological information from haematoxylin and eosin staining (H&E) with genomic, transcriptomic, or proteomic information to investigate relationship between cellular and molecular behaviour at different resolution levels.
The Three Levels of Data Integration
“Data integration, or data fusion, has three different levels that you can consider,” said Dexter. “You’ve got what’s called low-level, which is basically combining raw data together and then processing that as one whole dataset.” This provides a reduced representation of the whole dataset. Dexter cautioned that there were different datasets to consider across different data levels.
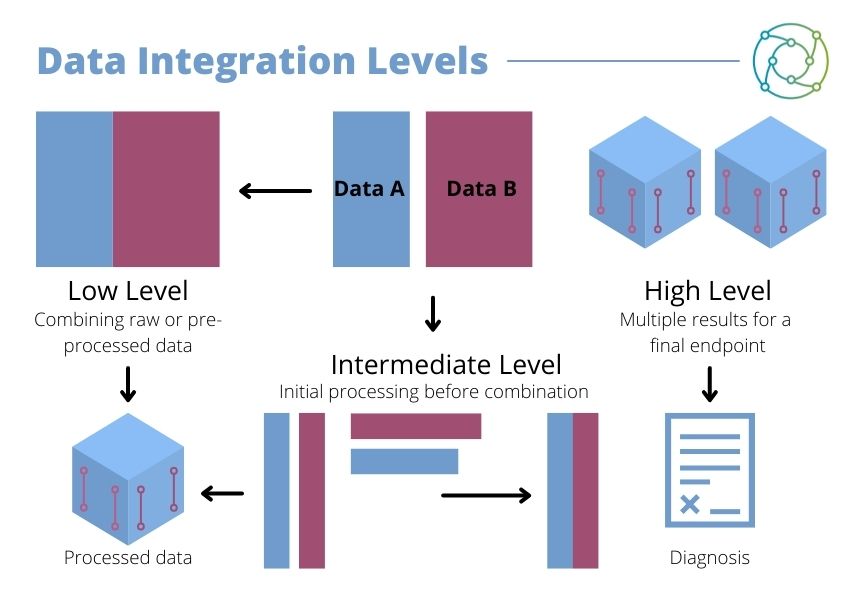
Some datasets may be combined straight away in low-level data integration (as seen in Figure 1), where raw or pre-processed data is combined. Others may be subject to an intermediate level of processing, with some initial processing applied before the data is combined. High level integration involves separate datasets being processed individually, then integrated afterwards.
A third approach is high level integration. This involves separate datasets being processed individually, then integrated afterwards with multiple results for high endpoint. Different approaches to data fusion have different applications in multi-omic analysis. “In spatial metabolomics and mass spectrometry imaging, one of the biggest challenges is how to identify what molecules are from a given peak,” said Dexter.
Approaches to Therapeutic Modalities
As Dexter clarified, the validation or confirmation of molecular identity depends on which kind of data integration studies researchers want to run. The combination of transcriptomic and genomic information across different modalities requires considerations to be made. Some datasets may be combined straight away with the combination of raw or pre-processed data, while others may be subject to an intermediate level of processing. Here, there may be some initial processing before the data is combined.
- Sparsely Connected Autoencoders: A Multi-Purpose Tool for Single Cell Omics Analysis
- Mass Spectrometry: Modern Medicine’s Swiss Army Knife
- Increased Yields and More Efficient Workflows for Extraction from FFPE
Providing a comparison of statistics from spatially resolved regions in MSI to bulk LC-MS/MS information, Dexter explained that the tandem approach to sequencing can provide accurate molecular information, while MSI can provide accurate statistical information specific to the tumour. Different molecules are identified as being highly differentiated.
The analysis of MSI images is guided by H&E, with the annotation of different types of data subject to image types. By combining various kinds of information, researchers can create a specific metabolic region. Another approach is the integration of MSI with histochemistry, along with different genetic models of cancer. “This can be done for different histochemical regions,” Dexter explained, “so when you see changes you know you’re not just seeing artefacts left from the data but trends within the tumour.”
Combining Mass Spectrometry Imaging with Transcriptomics
“You don’t always need to combine the two datasets together,” said Dexter, “but the combination can be used to get more information about a disease, more drug targets, and better drug targets.” This does not necessarily mean that multi-omics analysis must be run in tandem: the case is more that of combining different datasets to obtain more valuable data and data insights. The integration of data from different analytical techniques can be used to find or define new biomarkers, phenotypes, and druggable techniques for these methods.
“The combination of datasets can be used to get more information about a disease, more drug targets, and better drug targets.”
As Dexter explained, “the fundamental differences are in the different types of data that you get.” Attempting to combine mass spectrometry with genomics underlines the huge difference in both technique and sequencing approach. Dexter articulated this difference by breaking down different omics sequencing techniques into two subsets: mass spectrometry-based techniques with a molecular structural dynamic, and sequencing-based subsets focused on data integration.
There exists a significant barrier to integration between these two approaches due to the different natures of the complexities involved in each. “Every layer you go across this buffer increases the complexity of this overlap,” added Dexter.
Fusion Datasets and the Potential of Higher Quality Treatment
There are also endemic challenges involved with bridging between sequencing-based omics and mass spectrometry-based omics. Each increase in integration brings small jumps across datasets, with low-level fusion evident for MSI. Fusion datasets, in certain circumstances, will always be driven by the data that is larger, as Dexter explained. “This is something that we’re actively working on right now, but when you see multi-omics, it’s not always a case of simply combining datasets.”
Dexter rounded off his presentation by asserting that spatial metabolomics can provide a wealth of information. When used in combination with other techniques, the quality of this information can be improved. Intermediate-level fusion techniques can help to augment resolution in spatial metabolomics, while the combination of experimental and computational workflows can be used to combine spatial metabolomics with transcriptomics. Low level fusion of this data is challenging on account of scaling and scalability, but the potential for higher-quality treatment is huge.
Want to read more about the latest advances in multi-omics analysis? Head over to our Omics portal for insights from the industry's best and brightest. If you'd like to register your interest in our upcoming Spatial Biology US: In-Person event, visit our event website.