Machine Learning Methods in Drug Delivery: Three Approaches to Watch
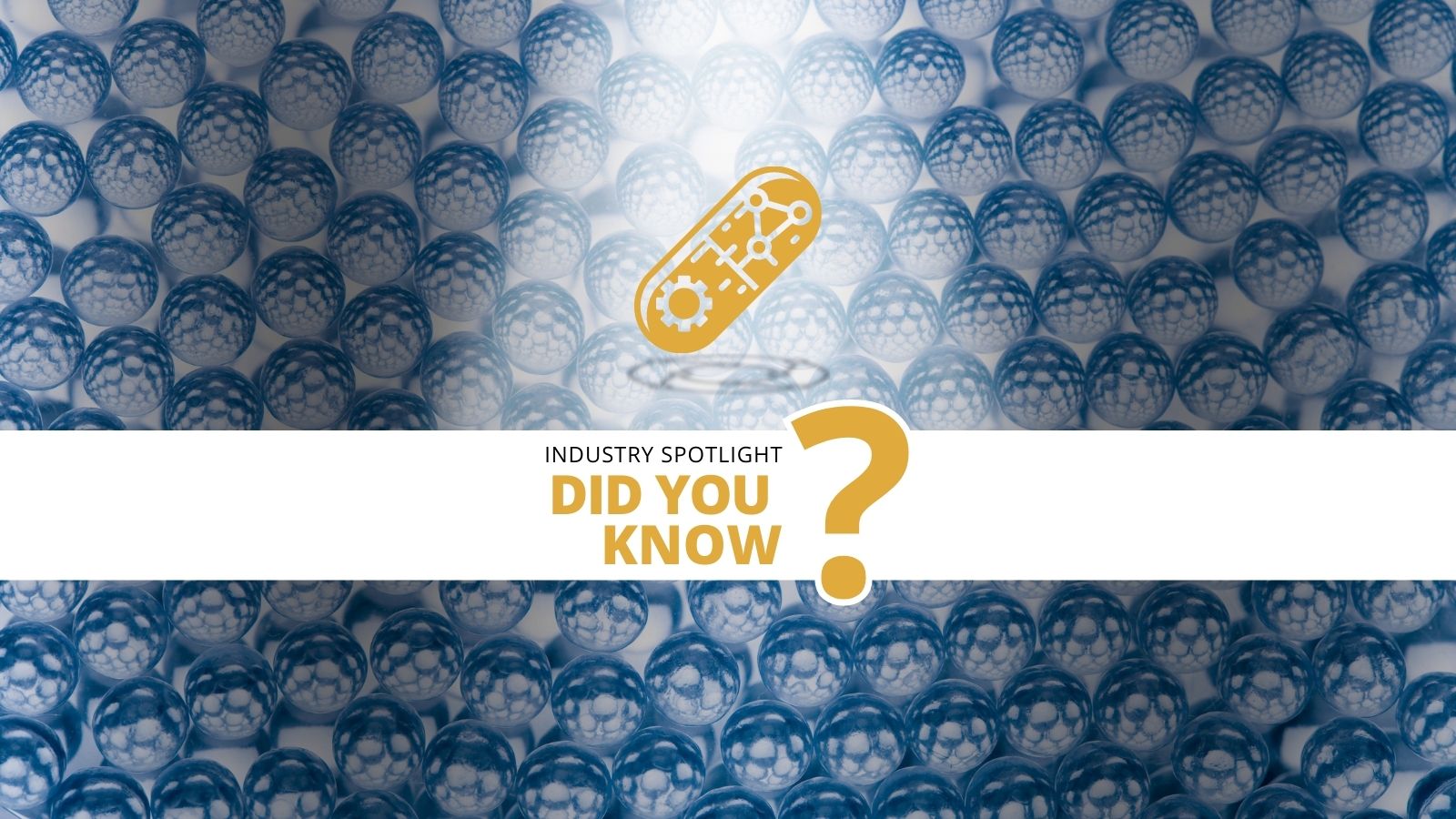
Machine learning methods have a plethora of applications in drug delivery, with the opportunity for significant improvements in prediction accuracy.
Some key focuses presently being pioneered in both industry and research include predicting drug loading in particulate matrices, anticipating the permeability enhancement through body barriers, and capturing stable drug delivery systems through different carriers and matrices.
Machine Learning used to Find Nanoparticles with High Drug Loading Capabilities
At MIT, a team of researchers have used machine learning to identify pairs of small-molecule drugs and inactive ingredients that will self-assemble into nanoparticles with high therapeutic payloads.
While nanoformulations of therapeutic drugs are transforming the effective delivery and treatment of a range of diseases, these nanoformulations are often complex to produce.
Previous efforts to develop nanoparticle formulations have been held back by an inability to predict which mix of ingredients will have high loading capacities.
Machine learning methods have been used with high-throughput experimentation to explore ways of circumventing this constraint.
The project evaluated 788 therapeutic small molecules, as well as 2,686 approved excipients through the rapid and large-scale identification of nanoformulations.
Regarding potential additional applications for the technology, the platform could one day propose personalised sets of excipients for patients with allergies to particular ingredients.
Predicting Drug Permeability across the Blood Brain Barrier
A common obstacle for the development of many therapeutics, the blood-brain barrier (BBB) is largely impermeable to most typical drug compounds.
Developers looking to synthesise a treatment targeting the brain — particularly in the context of central nervous system (CNS) drug discovery — need to think outside the box.
With massive increases in compound databases being recorded, machine learning methods and deep learning approaches have shown great promise in developing drugs that can cross the BBB.
A team of researchers from the University of Electronic Science and Technology of China have been investigating leveraging deep learning models for drug development.
Their research focus has been on training models to distinguish between drugs that are BBB permeable and those that are BBB impermeable, with the aim of integrating this approach into future drug discovery and development.
Anticipating Membrane Permeation of Drug Molecules
Further to this, a collaboration between researchers from Michigan State University and the University of Maryland in the USA are looking at combining implicit membrane models with machine learning to predict drug permeability.
Regardless of how a drug is administered, it will need to transverse lipid bilayer membranes during transport.
- Related: Where are we with inhaled biologics?
Among small and uncharged molecules, passive diffusion driven by a concentration gradient is the predominant method of membrane permeation.
The rate of permeation through lipid bilayer membranes is a critical metric for determining the overall efficacy of a drug.
Machine learning methods are applied in this context to increase the accuracy of predictions for experimental permeability coefficients.
Get your weekly dose of industry news and announcements here, or head over to our Formulation portal to catch up with the latest advances in manufacture and therapeutic delivery.