How AI Integration is Optimising Drug Development Pipelines
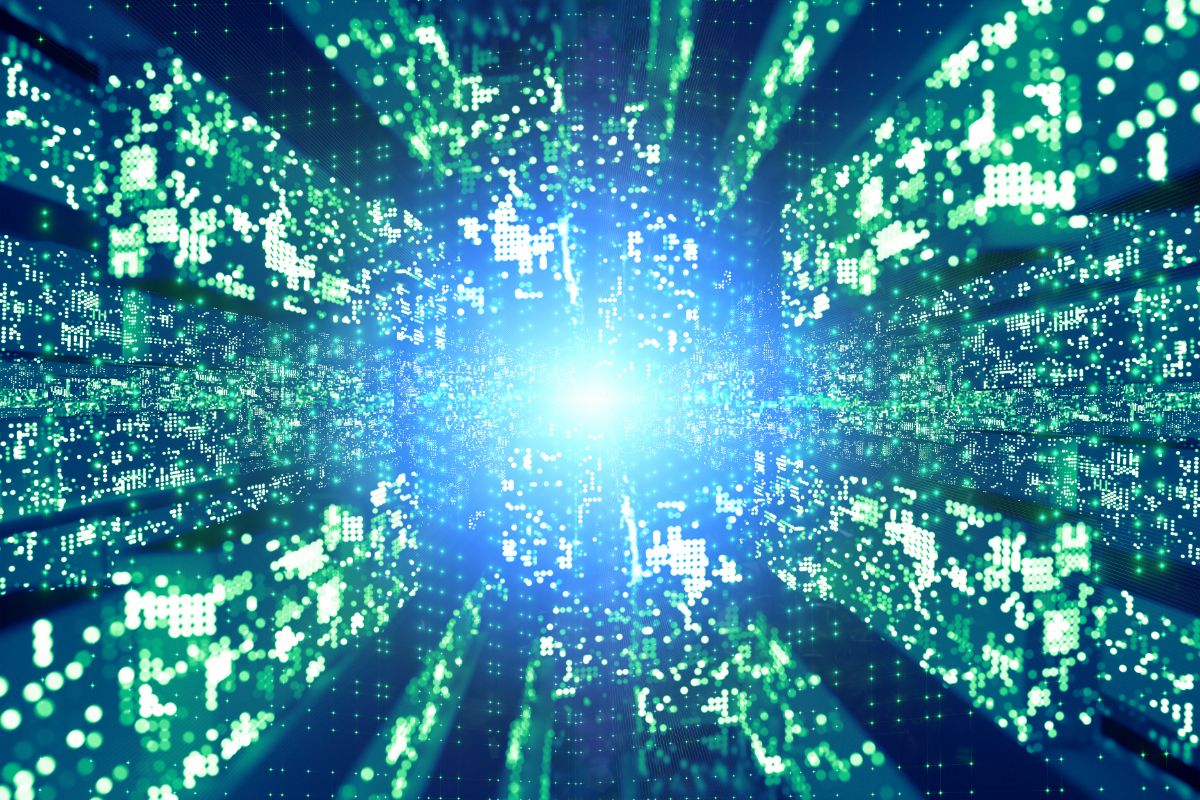
There are a wide range of roles that AI might play in drug discovery, from drug design and chemical synthesis to drug screening and repurposing. AI integration can also improve the process of decision-making and holds a lot of potential in the field of personalised medicine development.
At Oxford Global’s Pharma Data and Smartlabs UK: In-Person event in 2022, a panel of key opinion leaders from Genentech, Ipsen, and Horizon Therapeutics gathered to examine how the implementation of AI models is changing the landscape of drug development. They discussed the importance of reliable data and explainable AI when integrating machine learning models into drug discovery. They also explored where they believe the field will progress in the coming years, whether it be towards using AI for image analysis or simply improving the decision-making processes in drug development pipelines.
Meet the Panel
Hongmei Huang was Vice President of Development Sciences Informatics at Genentech. In this role, she was responsible for the strategic leadership around data management, informatics systems and analytics platforms for translational research and development functions. She also served on the leadership team for the Roche-wide effort to make data FAIR across R&D. With over 20 years' experience in the field, Huang has always focused on improving the success rate of clinical trials, and believes that AI holds the key to achieving this.
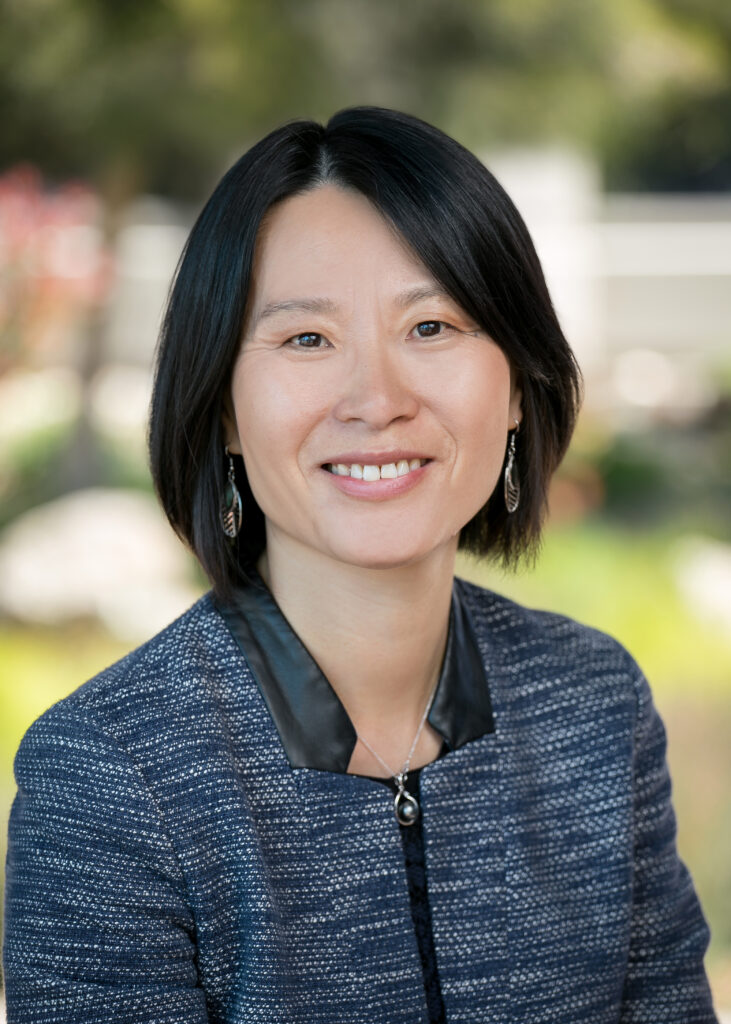
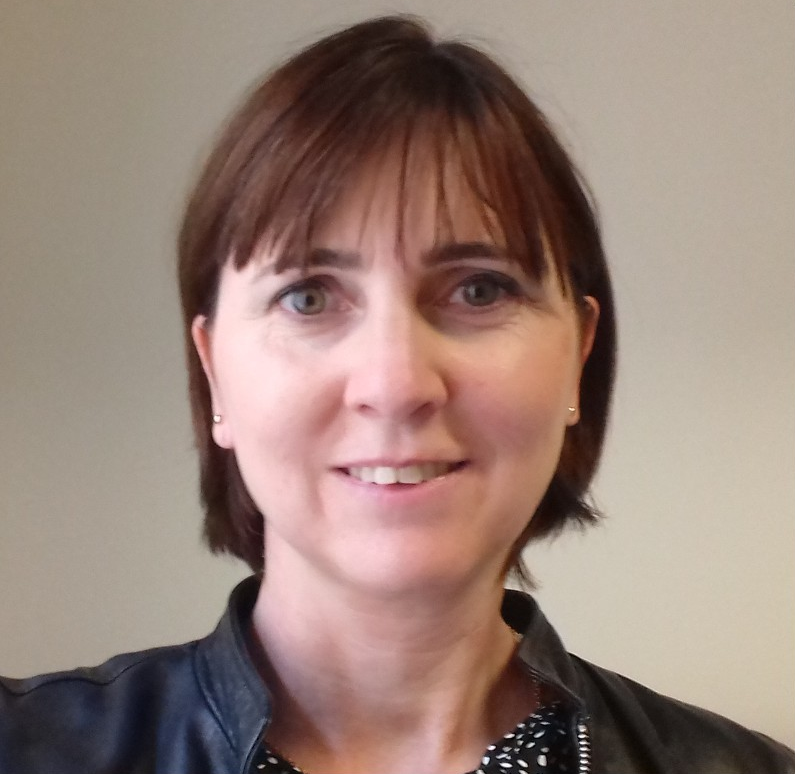
Maria del Pilar Schneider is a Senior Data Mining Statistician at IPSEN Innovation. She performs and supports R&D projects related to data analytics on Oncology, Rare Diseases, and Neuroscience by combining the use of Real-World-Data, Clinical Trial Data with innovative statistical methods, such as machine learning. She works closely in collaboration with company stakeholders such as Medical Affairs and HEOR.
Igor Rudychev, Vice President of Enterprise Analytics at Horizon Therapeutics, has over 25 years of data, analytics, and AI/ML experience. In the pharma field, he has worked on applying AI, ML, Experts Systems, and Advanced Analytics to R&D, Labs, Medical, Clinical and Commercial Pharma analytics. With a PhD covering applied mathematics and theoretical physics, Rudychev presents and publishes extensively on new data & analytics methods in pharma.
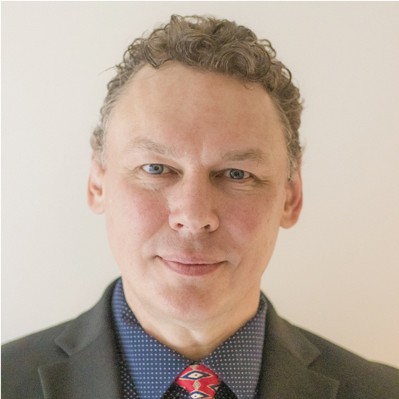
Reliable Data for Reliable Results
As the capabilities of AI models grow, they are progressively being introduced into decision-making processes throughout the drug discovery and clinical development process. However, training models to make reliable decisions depends on using real facts and reliable data. One audience member started the discussion by asking about the viability of using small datasets from more limited clinical trials to train AI/ML models.
Rudychev was quick to respond that even from a small trial of 12 patients, the data gained would be more than sufficient to apply to AI models. Indeed, as Schneider commented, small-data ML can be a valuable exploratory tool when working with limited data sets.
Huang agreed with these views and added that reframing the questions we ask about how data and AI interact may be a powerful approach when looking to integrate AI into the drug discovery and clinical development pipeline. In addition to attempting to implementing AI and ML tools into individual trials, Huang recommended also leveraging pre-existing data from multiple studies, as this is more likely to be reliable data.
Looking to utilise pre-existing data more effectively when training AI/ML models will aid in the process of patient stratification and how we understand the different responses which emerge in different demographics. Furthermore, looking at data in this way will influence clinical trial design to make them more targeted, and will move us closer towards personalised healthcare.
The Need for Explainable AI
Whilst AI/ML models can help enormously with the decision-making process, one pitfall researchers must be careful to avoid is complete dependence on these models. This is because full dependence often leads to decisions made by models becoming unexplainable, which causes issues when findings are presented to regulatory bodies.
- FDA Release New Guidance for Modifications to AI/ML-Enabled Devices
- Revolutionary Organ Transplant Selection with Artificial Intelligence
- AI/ML Approaches in Drug Development: Applied Machine Learning in Clinical Trials
Huang noted that regulators will always require at least some drug safety data, and therefore researchers must be able to understand and explain the AI models’ decision-making process during drug development. Researchers in the field are keenly aware of this requirement. One audience member commented that natural language generation is widely used to ensure that AI models are explainable. The ultimate aim is that a layperson, including regulators or patients, will be able to understand the decisions made by AI models.
Future Opportunities for AI Integration in Drug Discovery and Clinical Development
The potential of AI to revolutionise the drug discovery pipeline is vast, and the panel were keen to share their thoughts on the outlook of AI integration. Schneider expects to see a shift towards in silico clinical trials, which will use virtual patients and predictive toxicology.
She also thinks that AI will play a significant role in image analysis for neuroscience, noting that “the technology has the capacity to detect smaller elements which the human eye cannot see” and that this will therefore improve diagnosis.
Huang agreed with this outlook regarding the development of image analysis using AI models. More broadly, she thinks that as the tech and pharma sectors become more integrated, AI’s capabilities will become more sophisticated and will be able to solve more challenging problems in the drug development space more efficiently.
As models become smarter, they will be able to use the data we already have more effectively and provide more astute insights. Rudychev also referenced the fact that, as these models become smarter, we will see an increase in automation. As human knowledge is gradually built into AI models, the predictions and decision-making process that AI can bring to drug development pipelines will continue to improve. Huang closed by expressing how she now believes that we are at an inflection point in this field, saying “let’s think bolder, let’s take some risks, and let’s keep driving forward.”
Want to read more about how the AI integration will influence the landscape of drug development? Head over to our PharmaTec portal to see the latest insights from the industry’s best and brightest. If you’d like to learn more about our upcoming Pharma Data & Digital Medicine conference, visit our event website to download an agenda or register your interest.