AutoPrognosis, An Innovative Tool Used to Predict Lung Cancer Risk
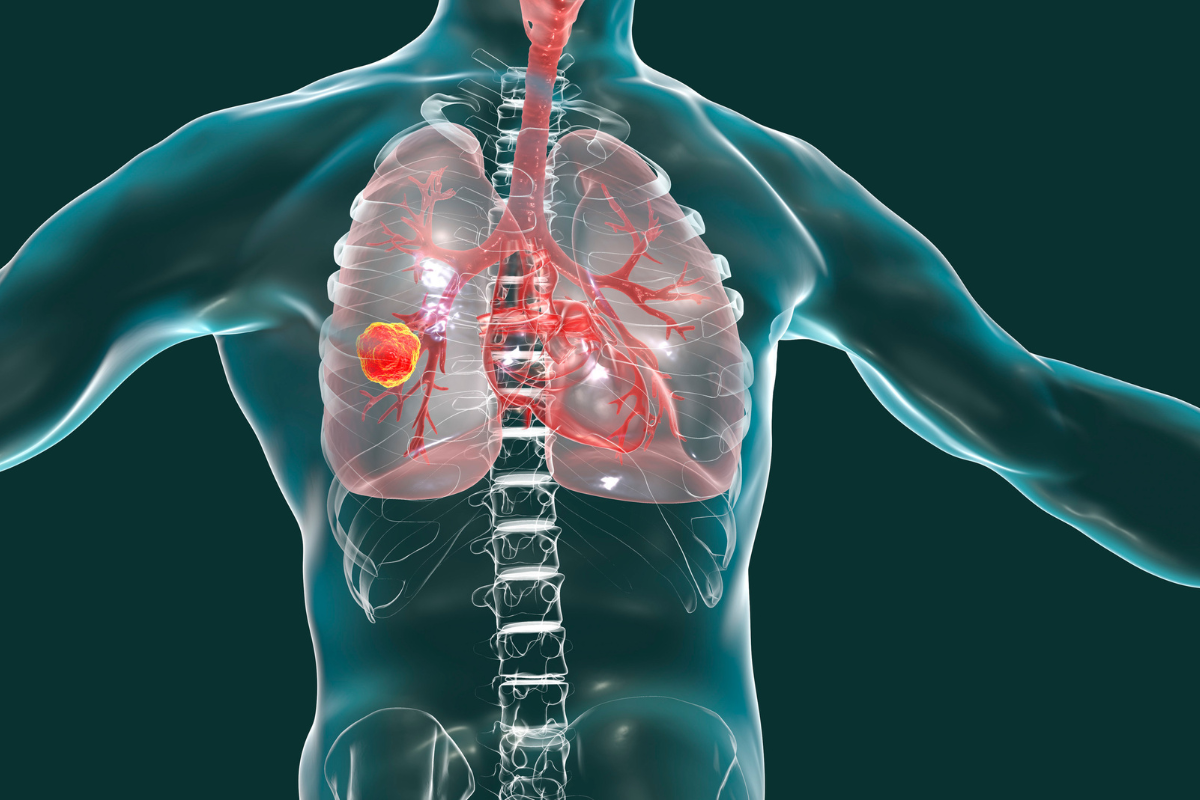
Researchers at University College London (UCL) and the University of Cambridge developed a tool, AutoPrognosis, that used machine learning (ML) to create predictive models for clinical prognosis. AutoPrognosis assessed lung cancer risk and boasted equivalent accuracy and clinical relevance using just a quarter of predictors when compared to existing models.
RELATED:
- Alphabet’s Calico and Terray Tx Announce Partnership to Tackle Age Related Disease
- Using ML with DEL: Philogen and Google Partner in New Study
- From Theory to Therapy: Accelerating Ligand-Based Virtual Screening with AI and ML Technologies
According to a study published by PLOS Medicine, AutoPrognosis demonstrated higher levels of accuracy in predicting an individual’s risk of developing lung cancer within the next five years compared to current risk models.
Drawing conclusions from the UK Biobank and the US National Lung Screening Trial, AutoPrognosis, analysed data from 216,714 ever-smokers (UK Biobank) and 26,616 high-risk ever smokers (US National Lung Screening Trial). Lung cancer risk was calculated based on just 3 variables in these data: age, number of years the subject spent smoking, and average number of cigarettes smoked per day. Despite using a small number of predictors, AutoPrognosis’s precision and prediction capabilities exceeded that of current conventional models.
Mihael van der Schaar, a Professor at the University of Cambridge, emphasised the system’s impressive application, by stating, “While AutoPrognosis has already been applied for risk prediction and prognosis in numerous diseases, this is the first time it has been used to determine the minimal information needed to screen patients.”
Lung cancer is the leading global cause of cancer-related death. In 2020, lung cancer was responsible for 1.8 million deaths worldwide. Early detection is critical for improving survival rates; according to the National Cancer Institute, screening high-risk subjects for lung cancer had the potential to reduce lung cancer-specific mortality by 20 – 24% amongst those screened.
While the results of this study are highly promising, it is crucial to note that the data sets in the study only originated from the USA and UK. To account for the genetic and molecular makeup across a wide range of populations and geographical locations, data would need to be collected from other sources. This would add to the richness of the data sets, thus enhancing the model’s reliability and generalisability.
However, accessing data from a variety of different sources presents its own challenges. Data privacy concerns remain a controversial topic and patient confidentiality limits the availability of data which means the full potential of the model may not be fulfilled.
These findings show the potential to simplify screening processes for other cancers. For example, an advanced machine learning (ML) model developed by the Massachusetts Institute of Technology (MIT) outperformed current methods in detecting pancreatic ductal adenocarcinoma, the most common form of pancreatic cancer. Furthermore, this modelling approach could be applied to other diseases such as cardiovascular disease and chronic kidney disease.
With the demand for personalised medicine and patient-centric approaches becoming increasingly critical and prevalent, this case study demonstrates that we are one step closer towards achieving early prognoses of lung cancer, potentially reducing the number of lung cancer related deaths.