Unveiling the Essential Criteria for Validating Clinical Biomarkers: A Comprehensive Guide
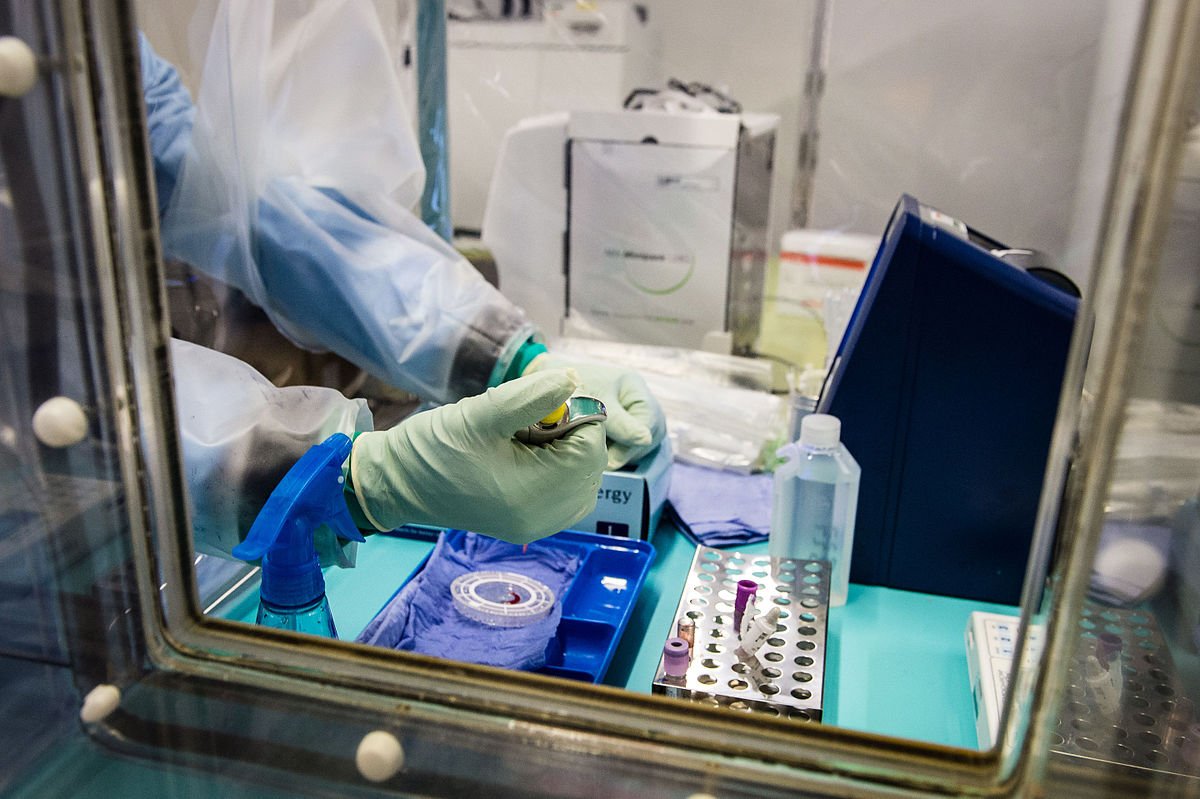
Clinical biomarkers play a crucial role in modern medicine, enabling early detection, diagnosis, and monitoring of diseases. These biomarkers, which can be molecules, genes, or characteristics measured in biological samples, provide valuable insights into a patient's health status and response to treatment. However, the development and validation of clinical biomarkers is a complex and rigorous process. Biomarkers by Oxford Global will explore the essential criteria for validating clinical biomarkers, shedding light on the steps and challenges involved.
Importance of Validating Clinical Biomarkers
Validating clinical biomarkers is vital to ensure their accuracy, reliability, and clinical utility. Without proper validation, biomarkers may lead to misdiagnosis and ineffective treatments. The validation process establishes the link between the biomarker and the target disease or condition, providing scientific evidence of its validity and clinical relevance. Validated biomarkers also enhance the efficiency and cost-effectiveness of clinical trials, enabling researchers to identify patients who are more likely to respond to specific treatments. Ultimately, validating clinical biomarkers paves the way for personalized medicine and improved patient outcomes.
Criteria for Validating Clinical Biomarkers
Validating clinical biomarkers involves a rigorous evaluation based on specific criteria. These criteria ensure that the biomarker is accurate, reproducible, and clinically meaningful. The following are the essential criteria for validating clinical biomarkers:
- Analytical Validity
Analytical validity refers to the biomarker's ability to accurately and reliably measure the target molecule or characteristic. It involves assessing the biomarker's sensitivity, specificity, precision, and accuracy. Sensitivity determines the biomarker's ability to detect true positive cases, while specificity measures its ability to exclude false positive cases. Precision reflects the biomarker's consistency in producing the same result under different conditions, and accuracy determines how closely the biomarker's measurements align with the true value.
- Clinical Validity
Clinical validity evaluates the biomarker's ability to identify and predict the presence or absence of a specific disease or condition. It involves assessing the biomarker's sensitivity, specificity, positive predictive value, and negative predictive value in a clinical setting. Sensitivity and specificity remain crucial in clinical validity, as they determine the biomarker's ability to correctly identify true positive and true negative cases. Positive predictive value measures the probability of having the disease when the biomarker is positive, while negative predictive value measures the probability of not having the disease when the biomarker is negative.
- Clinical Utility
Clinical utility focuses on the practical application of the biomarker in clinical practice. It assesses whether the biomarker provides valuable information for clinical decision-making, patient management, and treatment selection. Clinical utility considers factors such as the biomarker's impact on patient outcomes, cost-effectiveness, and feasibility of implementation. A clinically useful biomarker should demonstrate improved patient outcomes, facilitate targeted therapies, and provide a clear benefit over existing diagnostic or prognostic methods.
Types of Clinical Biomarkers
Clinical biomarkers encompass a diverse array of molecules, genes, and characteristics measurable in biological samples. Understanding these variations is pivotal for their validation and subsequent clinical utilization.
Diagnostic biomarkers play a crucial role in disease identification, aiding in distinguishing between similar conditions or enabling early detection. Leveraging specific molecular or genetic changes associated with a disease, they ensure accurate diagnoses and prompt interventions. Prognostic biomarkers offer insights into disease progression and outcomes, informing personalized treatment plans and determining therapeutic intensity. Predictive biomarkers further refine treatment strategies, identifying patients likely to benefit from specific therapies while sparing others from unnecessary interventions. Additionally, pharmacodynamic biomarkers shed light on a treatment's biological effects, guiding drug development and optimizing patient care through tailored therapies and efficacy monitoring.
Common Challenges in Clinical Biomarker Validation
Validating clinical biomarkers presents significant challenges that can impede their translation into clinical practice. Various factors complicate the validation process, requiring careful consideration and robust strategies to overcome obstacles. Among the common challenges faced during clinical biomarker validation, sample collection and storage stand out as crucial aspects. Obtaining high-quality biological samples and ensuring their proper preservation is essential for accurate validation. Standardizing procedures for sample collection, processing, and storage is imperative to minimize variability and ensure reliable biomarker measurements.
Additionally, the inherent heterogeneity and variability within diseases and patient populations pose significant challenges. Factors such as genetic diversity, disease subtypes, environmental influences, and co-existing conditions can impact biomarker performance, necessitating large and diverse study cohorts for validation. Addressing these complexities requires meticulous statistical analyses and robust validation strategies to ensure the reliability and generalizability of biomarker findings. Furthermore, reproducibility is fundamental in biomarker validation, yet it faces obstacles due to the lack of standardized protocols and variability in assay platforms. Establishing rigorous quality control measures, standardizing assays, and conducting inter-laboratory validation studies are essential steps to enhance reproducibility and facilitate the clinical application of biomarkers.
Tools and Techniques for Clinical Biomarker Discovery
The discovery of clinical biomarkers relies heavily on advanced tools and techniques that enable the precise identification and characterization of relevant molecules or characteristics. These tools are instrumental in the initial phases of biomarker development and validation, providing researchers with the means to navigate the intricate landscape of biological systems. Genomic and proteomic technologies take precedence in this endeavour, offering comprehensive analyses of genes, proteins, and their interactions. Techniques like next-generation sequencing, microarrays, and mass spectrometry unveil genetic variations, gene expression patterns, and protein profiles, thereby shedding light on disease mechanisms, potential biomarkers, and personalized therapeutic targets.
In tandem with genomic and proteomic approaches, imaging and molecular imaging techniques play a pivotal role in biomarker exploration. Non-invasive visualization and characterization of anatomical structures and functional processes, facilitated by imaging modalities such as MRI, PET, and CT, are integral to biomarker discovery and validation. Molecular imaging techniques, including fluorescence imaging and radioactive tracers, enhance this process by targeting specific biomarkers or molecular pathways of interest. Beyond biomarker discovery, these imaging tools contribute to validating and monitoring treatment response, offering crucial insights into disease progression and therapeutic efficacy.
Case Studies of Successful Clinical Biomarker Validation
Examining case studies of successful clinical biomarker validation provides valuable insights into the practical application of validated biomarkers in clinical practice. The following are two examples of successful clinical biomarker validation:
- HER2 Biomarker in Breast Cancer
The HER2 biomarker is a protein overexpressed in certain breast cancer patients. Its validation as a predictive biomarker for response to HER2-targeted therapies, such as trastuzumab, has revolutionized the treatment of HER2-positive breast cancer. Clinical trials and extensive validation studies demonstrated that patients with HER2-positive breast cancer, as determined by immunohistochemistry or fluorescence in situ hybridization, have significantly improved outcomes when treated with HER2-targeted therapies.
- PD-L1 Biomarker in Immunotherapy
Programmed death-ligand 1 (PD-L1) is a protein expressed on cancer cells that can inhibit the immune response. The validation of PD-L1 as a predictive biomarker for response to immune checkpoint inhibitors, such as pembrolizumab, has transformed the field of cancer immunotherapy. Clinical trials showed that patients with PD-L1-positive tumors, as determined by immunohistochemistry, have higher response rates and improved survival when treated with PD-1/PD-L1 inhibitors.
Future Trends in Clinical Biomarker Validation
The field of clinical biomarker validation continues to advance, driven by technological innovations and increased understanding of disease mechanisms. Several future trends are shaping the future of clinical biomarker validation:
- Multi-omics Approaches
The integration of multiple omics data, such as genomics, transcriptomics, proteomics, and metabolomics, promises a more comprehensive understanding of disease processes and personalized medicine. Multi-omics approaches enable the identification of complex molecular networks, biomarker signatures, and therapeutic targets. Integrating diverse omics data sets will enhance biomarker discovery and validation, leading to more precise diagnostics and targeted therapies.
- Liquid Biopsies
Liquid biopsies, such as blood or urine tests, offer a non-invasive and real-time approach to biomarker detection and monitoring. These tests analyse circulating tumour cells, ctDNA, exosomes, or other biomarkers present in body fluids. Liquid biopsies have the potential to revolutionize cancer diagnostics, allowing for early detection, treatment monitoring, and detection of minimal residual disease or recurrence.
- Artificial Intelligence and Machine Learning
The application of artificial intelligence (AI) and machine learning algorithms in biomarker validation is gaining momentum. AI can process vast amounts of data, identify complex patterns, and generate predictive models. Machine learning algorithms can optimize biomarker selection, predict treatment response, and support clinical decision-making. AI and machine learning will play a crucial role in accelerating biomarker discovery and facilitating personalized medicine.
Conclusion
Validating clinical biomarkers is a critical step in translating scientific discoveries into clinical practice. The essential criteria for validating clinical biomarkers, including analytical validity, clinical validity, and clinical utility, ensure their accuracy, reliability, and clinical relevance. Overcoming the challenges in biomarker validation requires standardized protocols, diverse study cohorts, and rigorous quality control measures. Advanced tools and techniques, such as genomic technologies, imaging techniques, and bioinformatics, aid in biomarker discovery and characterization. Validated clinical biomarkers enable personalized therapy and improve patient outcomes. Looking ahead, future trends in clinical biomarker validation, including multi-omics approaches, liquid biopsies, and artificial intelligence, hold great promise for advancing personalized medicine and transforming patient care.