The Potential Uses of AI in Biomarker Discovery and Biomarker Validation
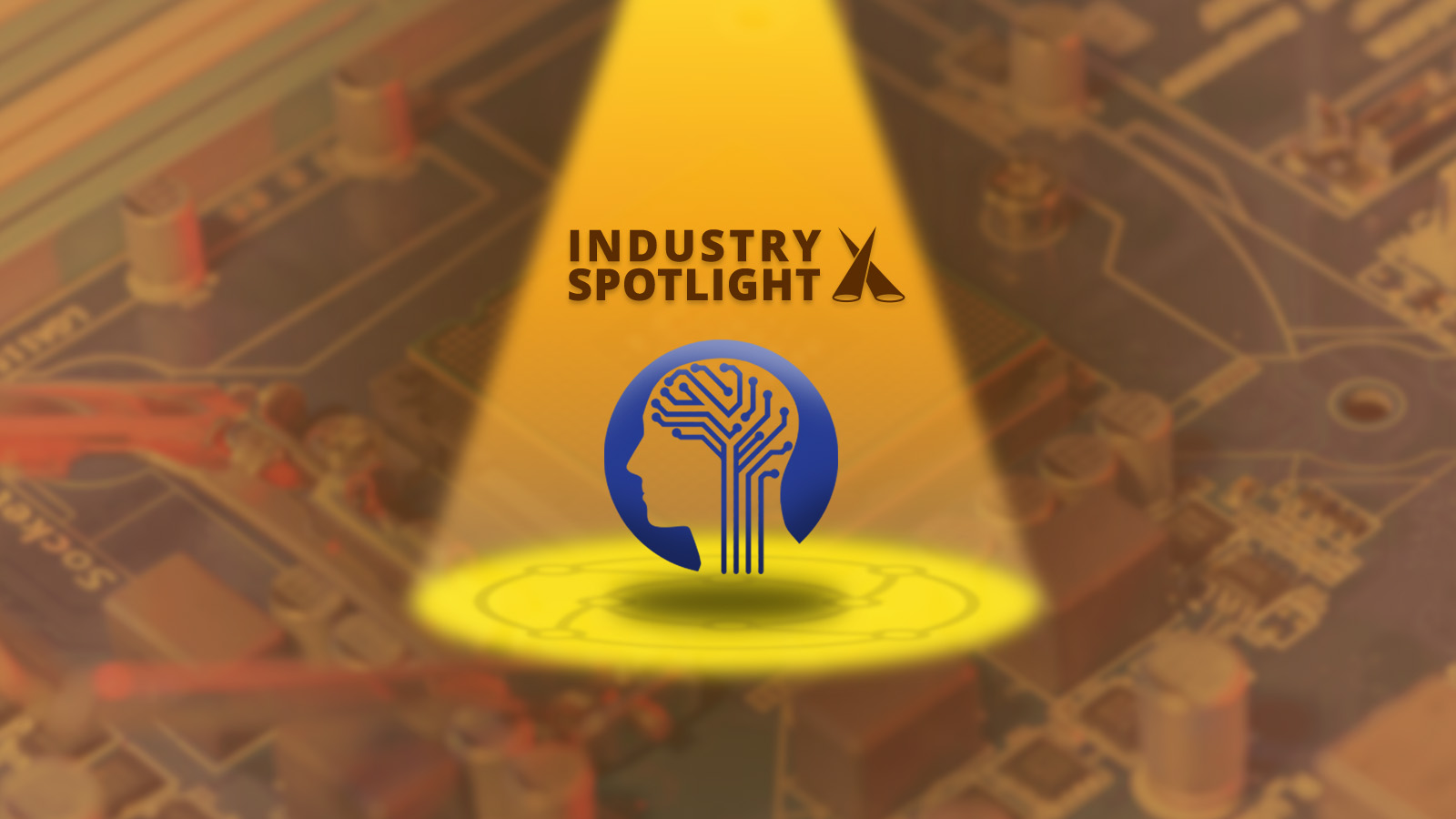
AI (artificial intelligence) has the potential to significantly accelerate the process of biomarker discovery and validation, streamlining drug development approaches.
AI’s applications in biomarker discovery were a central focus at Oxford Global’s Biomarkers 2023 conference, which covered many aspects of automation and machine learning currently being explored in the industry.
While the timeframe varies subject to the therapeutic area in question, it takes a little over ten years for a drug to progress from phase I development to regulatory approval.
Biomarkers have come to inform part of the early decision-making and proof of concept process for compound development.
- Did the coronavirus pandemic reshape international standards for therapeutics?
- Combining clinical biomarker findings with computational biology
- Novel therapeutic targets for breast cancer assessed in early-phase trials
Effective biomarker strategies can shorten the drug development process and bring down the overall time for approval by well over a year.
So how can AI support drug discovery and biomarker validation? The answer depends on the specific process being investigated and its aim.
One approach is the identification of diagnostic biomarkers to identify inclusion and exclusion criteria in development.
In this context, AI serves an auxiliary role in biomarker discovery as a means of investigating and cross-referencing the presence of particular biomarkers across a range of different datasets to validate a particular subset.
This approach to biomarker validation could be a great help with the issue of traceability: avoiding poor biomarkers which have previously been investigated and found to be nonviable.
Real-World Applications of AI in Biomarker Discovery
However, real-world applications of AI discovery can be a little more complicated than just introducing a new approach to biomarker validation.
The introduction of automated processes that aim to streamline identification and discovery can also make this work more complex.
Any data generated from this process will itself require validation and interpreting, which — while the procedure is being implemented and properly established — could be as time-consuming as the original procedure.
With the explosion of data available, researchers are already pressed to stay up to date on scientific developments.
While AI-led approaches to stratification of data could help in the initial stages of biomarker research around the development of a new therapeutic, implementation of a specific strategy would hinge on this not increasing the work burden.
Still, the promise shown by the use of AI to push the development of imaging biomarkers shows great promise for improving disease understanding.
With efforts already being made in certain clinical environments to apply AI to imaging data for improving biomarker validation, the field is guaranteed to see more instances of automation being used to help in development.
The onus will be on the developers to make sure these technologies can be implemented without hindering the overall development process.
Get your weekly dose of industry news and announcements here, or head over to our Biomarkers portal to catch up with the latest advances in cellular therapies.