Lung Cancer Patient Response to Immunotherapy Could be Predicted Through AI-Calculated Biomarker
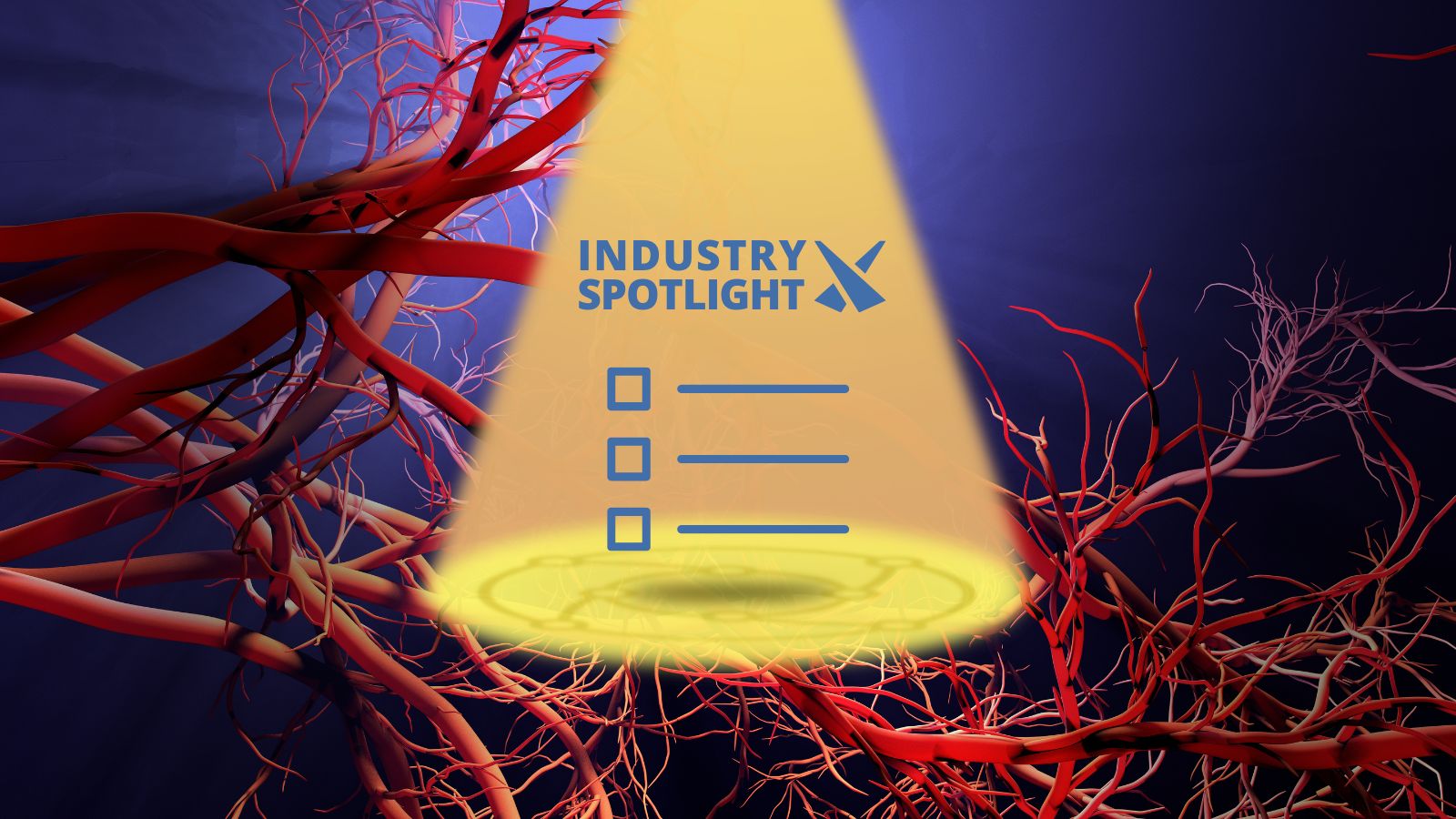
Lung cancer patient response to immunotherapy could be predicted through the use of an AI-calculated biomarker, according to Fierce Biotech.
A collaborative group of US researchers from institutions including Emory University, Cleveland Clinic, and NYU Langone Health trained an algorithm to comb through scans of non-small cell lung cancer (NSCLC) tumours.
The aim was to prospect for specific indicators of how well an individual may respond to immunotherapy treatments.
In the clinic, immunotherapy is often the first treatment option for NSCLC — the most common form of lung cancer — despite it being prohibitively expensive, with success rates of less than 50% of patients.
Anant Madabhushi, Professor at Emory and Georgia Tech’s shared biomedical imaging department, said the current immunotherapy treatment only tends to benefit 30% of patients.
“With the high expense of treatment and a 70% failure rate, we have to find better ways to predict and monitor responses to therapy,” he said of the research.
Tortuosity as an AI-Calculated Biomarker
The new biomarker prospected by the research coalition has been dubbed quantitative vessel tortuosity (QVT), as it assesses the arrangement of blood vessels surrounding a tumour.
Vasculature associated with tumours is more twisted and chaotically arranged than normal blood vessels, enabling cancer cells to grow faster and spread more easily throughout the body.
Researchers used AI tools to examine these vessels in more than 500 NSCLC patients, both before and after they had been treated with immune checkpoint inhibitor (ICI) therapies.
From the findings, the study’s authors determined that tumour-associated vasculature is even more twisted in patients who respond poorly to treatment.
This is likely because anti-tumour cells have a harder time reaching the tumour in highly twisted vessels in lung cancer, lowering the likelihood of patient response to treatment.
Interpreting QVT for Lung Cancer Patient Response and Treatment
Following these observations, researchers were able to train a machine learning algorithm to analyse the blood vessels around a tumour using only a CT scan, calculating the likelihood of a patient responding to immunotherapy.
While the observed success rate only came slightly higher than blinded approaches — predicting treatment response with an average area under the curve of 0.65 across three test sets — the implications are positive for future applications.
With further development and validation in clinical trials, the QVT biomarker and AI calculator has significant potential for treatment.
The need to non-invasively determine lung cancer patient response before therapy is initiated becomes crucial.
Other avenues where AI is being used to better predict and develop biomarkers include biomarker discovery in heart failure and the prediction of experimental peptide measurements from amino acid sequences.
Improvements in AI have made the interpretation of large datasets much easier, aiding clinicians by identifying markers of risk and facilitating the observation of prognosis.
Get your weekly dose of industry news and announcements here, or head over to our Biomarkers portal to catch up with the latest advances in cellular therapies.
Related Events
For more on this topic, you may be interested in our upcoming 20th Annual Biomarkers & Precision Medicine Congress , or our Biomarkers Europe 2024Related Resources
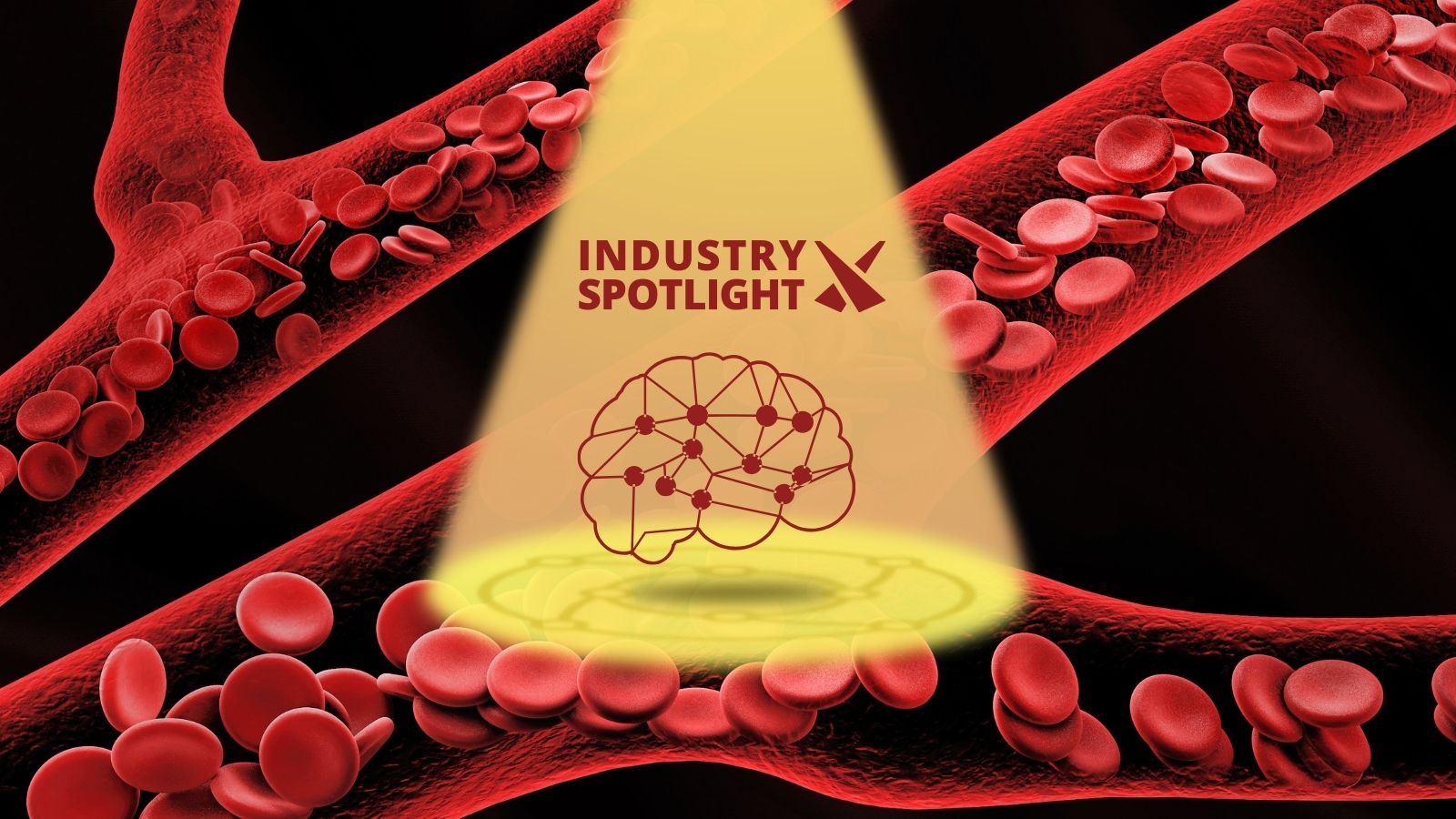