How Digital Biomarkers Can Enhance Pathologies and Patient Care
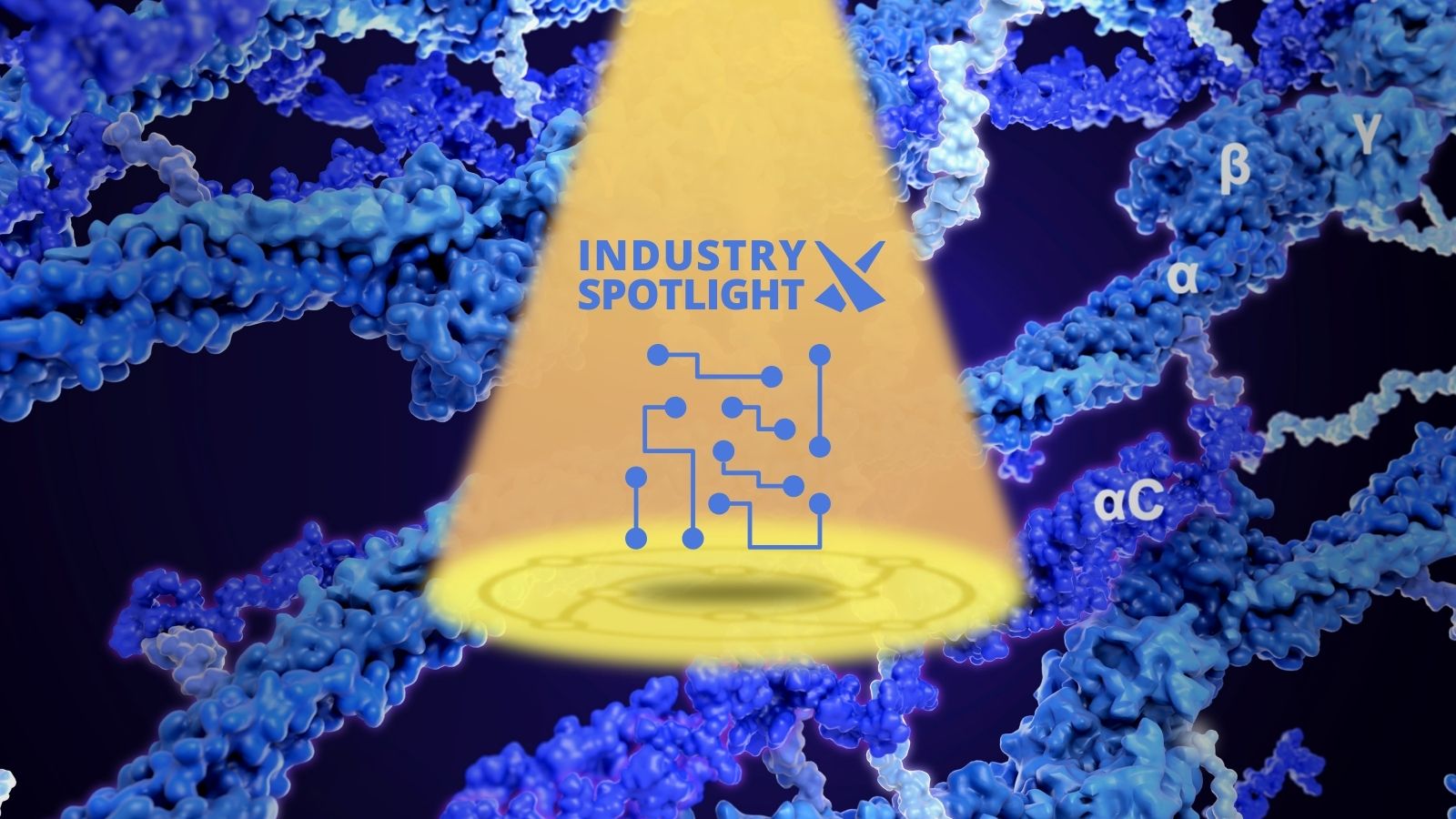
Digital pathology approaches could stand to substantially benefit from biomarker use for enhanced patient care, potentially ushering in a revolution in healthcare comparable to the mass uptake of genomics and gene modification approaches.
New technological breakthroughs will hopefully provide clinicians with more reliable performance metrics when dealing with rare cases which may otherwise be misidentified or overlooked.
Digital biomarkers – algorithms or patterns of data which correlate to a specific disease or condition – function as prognostic signals which can be used to detect signs of the presence or absence of a specific disease.
They can also be used to enhance clinical trial enrolment and patient assessments.
While they hold great potential utility, the adoption of digital biomarkers in healthcare will depend on the ease with which they can be integrated and their demonstrable cost-effectiveness.
As they involve the collection of multiple information points and data signatures, interpretation through machine learning techniques and approaches is often crucial.
Digital Biomarker Applications in Healthcare
Some of the biggest potential applications of digital biomarkers include the identification of rare diseases, which can often be misidentified.
While rare diseases by definition impact relatively few individuals, by aggregation they may affect more than 30 million patients across the US alone.
The implementation and broader availability of digital biomarkers can result in faster pathways to accurate diagnoses and more effective treatments.
This also avoids the spectre of misdiagnosis, which can result in healthcare costs of $USD 100bn per annum.
Potential applications of digital biomarker technology include the early diagnosis of diseases such as lung fibrosis, which can often see a two-year delay in diagnosis; in this time, a patient can lose a third of their lung function.
Reductions in cost are another benefit attached to digital biomarkers, avoiding expensive and invasive open surgery biopsies.
Given the scale and extent of chronic rare diseases in today’s healthcare ecosystem, taking advantage of advanced AI technologies and more proficient digital biomarkers is an essential step forward.
Potential Hurdles to Adoption and Uptake
While the adoption of digital biomarkers for improved disease prognosis is excellent in theory, in practice their implementation requires ample amounts of quality data.
To develop reliable models, thousands or even tens of thousands of dataset points and patients are needed: this is one of the most difficult hurdles to overcome when creating digital biomarkers.
In addition, there is no established FDA regulation regarding AI technologies with a specifically prognostic use case.
While the conversation is ongoing, widespread adoption will likely take several years, as all technical aspects need to be effectively integrated into existing IT systems.
There is undeniable potential in the field, with the mass uptake of genetics and genomics existing as a previous example of an experimental area which is now universally covered in clinical practice.
This promise is tempered with caution around the possibilities offered by AI and machine learning, as there is a lot that these technologies cannot achieve.
Still, enthusiasm for AI has hit new heights in the last few years, and understanding around digital biomarker solutions has never been greater.
Get your weekly dose of industry news and announcements here, or head over to our Biomarkers portal to catch up with the latest advances in diagnostics. To learn more about our upcoming Precision Oncology Europe conference, visit our event website to download an agenda and register your interest.