How AI is Changing the Game for Protein Design
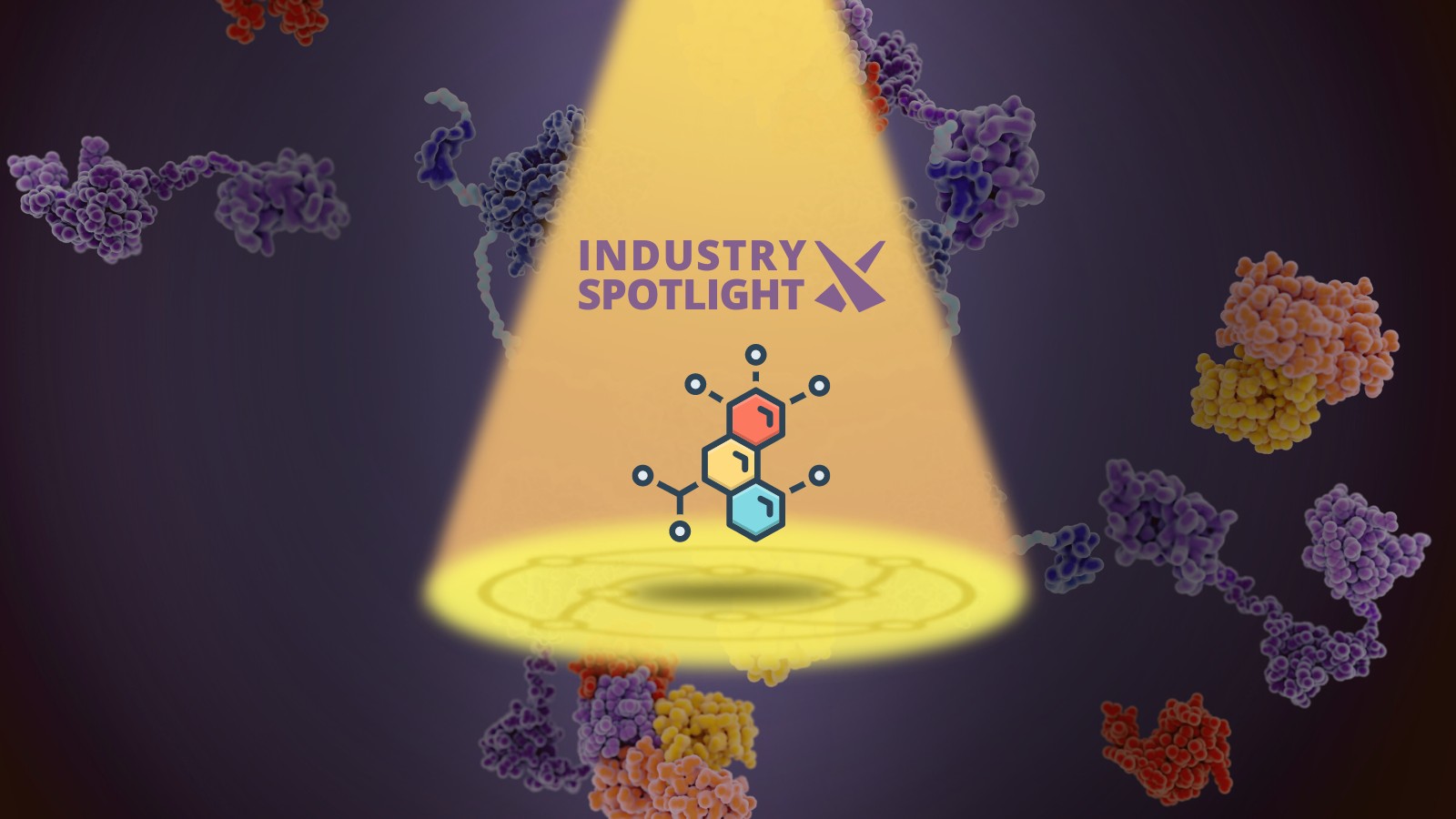
Artificial Intelligence (AI) has dramatically impacted how we understand and predict protein structure. You only need to look as far as DeepMind’s AlphaFold2 technology to appreciate that the field has changed entirely in recent years. Old protein structure prediction techniques such as x-ray crystallography, which are expensive, time-consuming, and require a high level of skill, are being replaced with more efficient and more effective AI algorithms. A team of researchers at the University of Washington, led by David Baker, have taken protein-folding AI a step further by using machine learning (ML) to synthesise artificial enzymes.
In an article published in Nature in February 2023, Baker and his team outlined how they had succeeded in using AI to design tailor-made, functional synthetic enzymes. Previously, protein engineering relied on making incremental changes to proteins which is a time-consuming and labour-intensive process; now, with AI, as Baker put it, “we can make even better designs more quickly than we could before.”
- The EMA’s Vaccines Working Party: The New Authority on Vaccines
- FDA Grant Approval for Alzheimer’s Drug Leqembi
- Biocon’s Biosimilar Difficulties: Successive FDA Rejections Don’t Inspire Hope
The researchers were looking to synthesise artificial enzymes called luciferases, a light-bearing enzyme widely used for bioassays and deep-imaging inside animal tissues. Very few luciferases are found in nature, as most are unstable and tend to bind to natural luciferins better than synthetic ones. These factors have previously inhibited attempts to create artificial luciferases and use them in scientific applications.
Baker and his team aimed to invent an amino acid sequence for a luciferase that could bind to synthetic luciferin and remain stable. Using ML, they predicted how well 4,000 proteins would bind to small molecules and identified nuclear transport factor 2 (NTF2) proteins as the most effective. However, amino acid loops in NTF2 proteins lead to issues with stability, so the team used ML to replace the loops with more stable amino acid combinations.
Working off of the foundation provided by NTF2 proteins, the AI algorithm produced 7,648 designs of proteins. Researchers then introduced these designs into E. coli bacteria to see which were functional, but only three met the mark.
Using this information, the research group narrowed down the number of candidate sequences and then conducted a second round of testing with a luciferase designed against another synthetic luciferin, which boosted their yield to 4% of 46 designs.
Baker said there was “still room for improvement” : the process was not fully automated, and manual sequence changes were required to perfect the synthetic enzyme’s active site. Baker also noted that the luciferase enzyme reaction is a fairly simple one to imitate, and therefore “we have work to do to see how well this approach works for more difficult chemical reactions.” Nonetheless, Baker is hopeful that one day AI will be able to synthesise artificial enzymes from start to finish without any human intervention.
Join Oxford Global’s annual Biologics UK: In-Person event today. This 3-day conference brings together a panel of prominent leaders and scientists, sharing new case studies, innovative data, and exciting industry outlooks.
Get your weekly dose of industry news here and keep up to date with the latest ‘Industry Spotlight’ posts. For other Biologics content, please visit the Biologics Content Portal.