FDA's AI Model Enhances Safety of Biosimilar Therapeutic Proteins
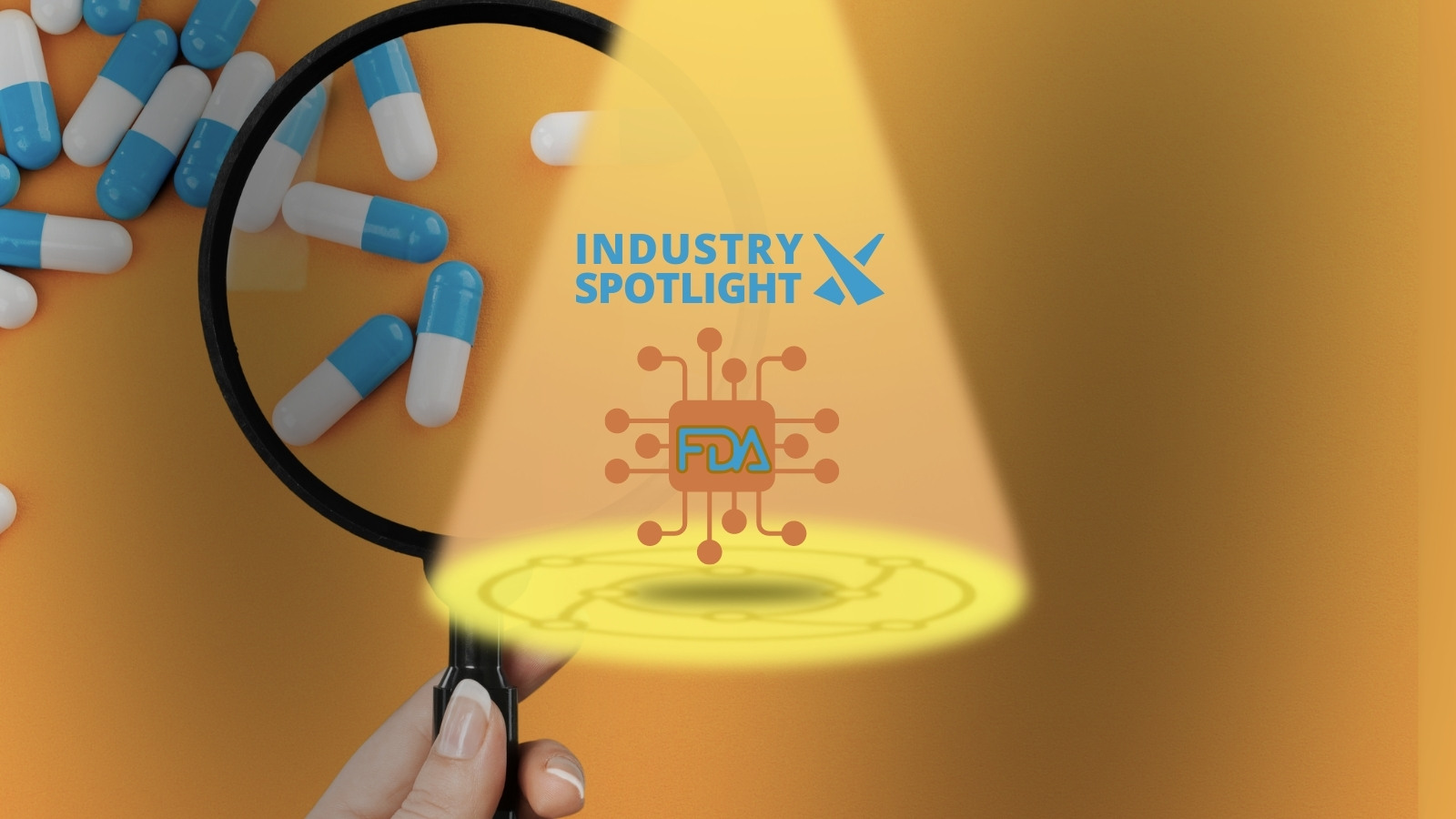
The FDA is at the forefront of scientific innovation when it comes to ensuring the safety and effectiveness of drugs. In a recent development, the FDA has revealed a new AI-based modelling technique to characterise protein aggregation, which significantly impacts the safety of biosimilar therapeutic proteins.
One major challenge that biosimilar developers face is characterising and comparing their products to reference products. This is particularly the case with regard to protein aggregation, which is caused under various stress conditions during manufacture, shipping, storage, and administration of a drug product. Even a minimal quantity of protein aggregates can trigger an immune response. Aggregation is challenging to monitor due to a wide variety of shapes, sizes, and compositions. Up until now, when developing biosimilar therapeutic proteins risk avoidance has been impossible.
In a recent study, the FDA used flow imaging microscopy to characterise aggregate protein particles. This imaging technique allowed them to record multiple images of single, subdivisible particles from a single sample. While these images contain lots of information about aggregate structure, manually extracting this information is time-consuming and often subject to human error, meaning that most of it is left unused.
- MIT Team Create AI-Generated Universal COVID Vaccine
- How AI is Changing the Game for Protein Design
- AI-Discovered Peptide has Dramatic Impact on Muscle Conditioning
To address this issue, the FDA applied an AI/ML approach which used convolutional neural networks (CNNs), a valuable tool for image analysis. This approach automatically extracts data-driven features encoded in images, such as fingerprints specific to stressed proteins. These features are then used to monitor the structural characteristics of particles in biotherapeutics and track the consistency of particles in a drug product.
The FDA used software called ParticleSentryAI to identify protein aggregation, anomalies during biological development and manufacturing processes, and batch variation. The AI model is trained using estimations of the most discriminatory parameters defined using images that are correctly labelled as either stressed or unstressed. Once trained, the CNN can predict which pre-defined labels best apply to a new image that has not been used in training.
ParticleSentryAI relies on the fingerprint approach, where the network is optimised to reduce the dimension of the spatially correlated image pixel intensities. This results in a new, lower-dimensional representation of each image. Extensive collections of images can be processed more efficiently and accurately than with traditional supervised learning approaches, as the technology can distinguish textural features that are not clearly defined within existing image processing software.
The findings of this study apply to a range of products in pharmaceuticals and biopharmaceuticals to monitor changes in product attributes during manufacturing, including protein aggregates. For developers working to produce biosimilar therapeutic proteins with a greater risk of protein aggregation, ParticleSentryAI will provide a more effective means to demonstrate biosimilarity and improve their products' safety profiles.
Join Oxford Global's Antibody Engineering: Online event today. An intensive 2-day meeting, it will delve into the latest in antibody engineering and antibody-based therapeutics, with a focus on AI/ML emerging technologies and antibody optimisation.
Get your weekly dose of industry news here and keep up to date with the latest ‘Industry Spotlight’ posts. For other Biologics content, please visit the Biologics Content Portal.