Computational Tools and AI/ML for Antibody Engineering
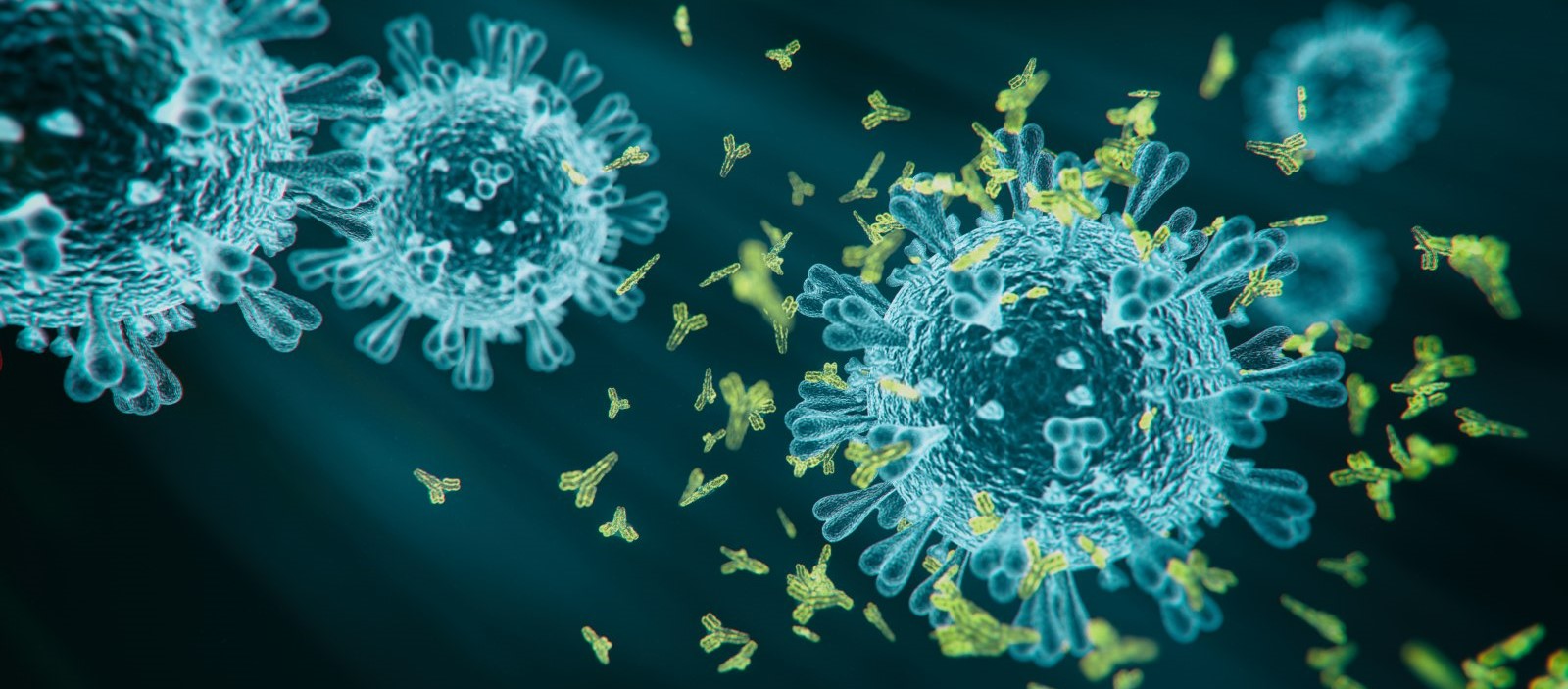
How are the ever-growing capacities of Artificial Intelligence (AI) and Machine Learning (ML) technologies changing the field of antibody engineering? On the first day of Oxford Global’s Antibody Engineering: Online event in 2022, representatives from Merck and AbbVie sought to offer some insight into how these companies are integrating new forms of AI/ML technology into their workflows for antibody discovery.
The online conference saw senior representatives from leading pharmaceutical companies and research institutions come together for three days of cutting-edge presentations, panel discussions, and live workshops in June 2022. Attendees heard about the current landscape of antibody engineering, market priorities, and challenges in the field.
One of the event highlights was the workshop at the end of Day One, which focused on adopting AI/ML in antibody engineering, with presentations by Shira Warszawski, Principal Scientist at Merck1, and Christopher Negron, Senior Scientist at AbbVie. In this insight article, we take a closer look at their presentations to bring you some key takeaways from their talks.
Presentation 1: Computational Tools In The Field Of Therapeutic Antibodies
Presented by: Shira Warszawski, Principal Scientist at Merck
Warszawski began her presentation with an overview of the major activities in antibody discovery. The process follows six steps before Phase I trials can begin: target identification and validation, hit discovery, hit optimisation, lead optimisation, exploratory development, and preclinical development (see Figure 1).
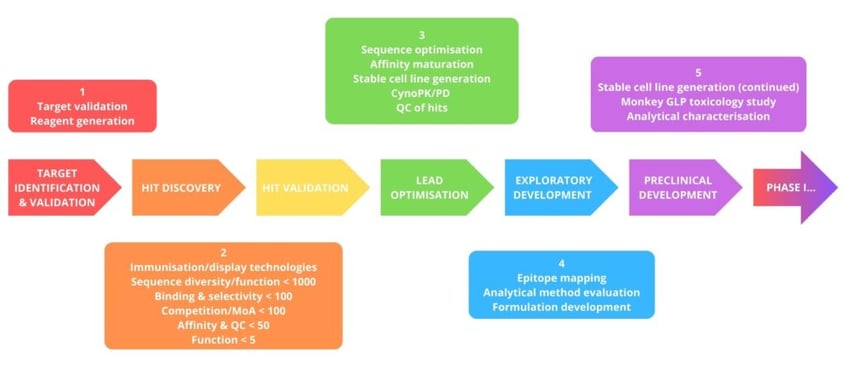
This antibody discovery process presents multiple challenges, many of which arise because it is difficult to ensure that candidates meet all of the criteria, including specificity, viscosity, ability, and solubility, amongst others.
The currently available computational tools used to predict antibodies are not particularly accurate, and there are also very few ML models for antibody discovery. Therefore, many pharma companies are now looking to integrate AI into their antibody discovery process to make it more robust and efficient. More specifically, these firms are looking to answer whether they can leverage AI to select and design better candidates earlier.
To illustrate how AI could improve the antibody discovery process, Warszawski focused on how Merck has tried to predict antibody clearance using AI/ML tools which can generate “more fully-optimised variants with improved biophysics and function.” The basic premise of this work was that, by inspecting the biophysical properties in vitro and in silico, antibodies with better linear clearance could be selected earlier on, meaning that researchers could predict how long these antibodies would remain in the body after dosing.
- AI-Discovered Peptide has Dramatic Impact on Muscle Conditioning
- The Future of Peptide Chemistry and Sustainability
- MIT Team Create AI-Generated Universal COVID Vaccine
Researchers initially established that the calculated values for clearance versus the published values fit the regression model. From there, they could assess the nature of the correlation between clearance and single biophysical properties. Researchers modelled these antibodies in MOE and calculated 23 biophysical descriptors. No statistically significant correlation was detected, suggesting that clearance is more complex than any single property as the biophysical factors which determine linear clearance are interdependent.
This research elucidated several of the challenges which arise when trying to predict antibody properties. There is a distinct lack of data on unsuccessful antibodies, as data is not publicly shared between companies. The nature of the antibodies themselves also raises issues, as they are very diverse, and each requires a different biological assay. Homology modelling is also not particularly accurate, regardless of the software used, and ultimately curating the data can be challenging too.
Increased collaboration between pharma companies will undoubtedly prove incredibly beneficial in overcoming these challenges. Warszawski pointed to the founding of AION labs, a new lab in Israel which is a collaboration between AstraZeneca, Merck, Pfizer and Teva to illustrate how data sharing may increase progress. Through this collaboration, the hope is that they will be able to develop an AI platform with an accurate library of antibodies which can bind to desired epitopes that can perform experimental validation more efficiently.
Presentation 2: Mitigating Off-Target Interactions of Affinity-Matured Antibodies via QSPR
Presented by: Christopher Negron, Senior Scientist at AbbVie
Computational chemists have long used quantitative structure-property relationships (QSPR) to find design strategies to improve the drug-like properties of small-molecule therapeutics. However, duplicating such efforts in the context of biologics modalities remains challenging. In his presentation, Negron discussed two case studies where QSPR was used in biologics drug discovery to improve the drug-like properties of affinity-matured antibodies.
At AbbVie, affinity maturation typically involves yeast display: they take an antibody that binds a known target and then make millions of variants. This often results in dozens of potential candidates, whose drug-like properties are then evaluated in several in vitro assays so that they can be prioritised. One of these assays measures off-target binding propensity or polyspecificity.
The two case studies assessed binding to HEK293 cells’, trying to answer the question, “is it possible to reduce off-target binding to HEK293 cells while retaining binding to the target?”
The first case study evaluated 28 affinity-matured variants that showed significant levels of HEK293 binding via FACS. Using a biologics-oriented QSPR workflow (see Figure 2), researchers observed that positive charge correlated strongly with HEK293 binding. The opposite was also true, negative charge inversely correlated with HEK293 binding.
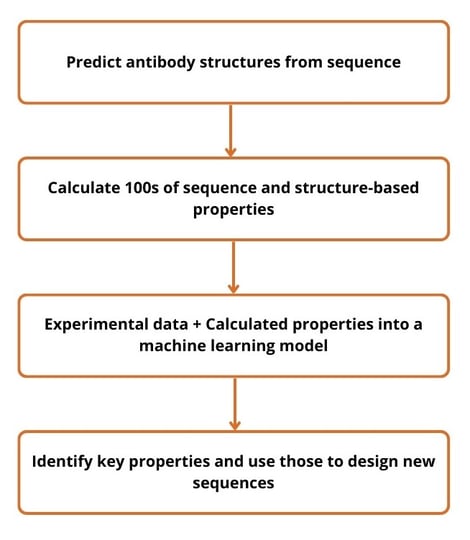
The second case study was designed to ensure that the results from the first project were not a one-off. Using the same technology and QSPR workflow, researchers again observed that positive charge correlated with HEK293 binding. Ultimately, Negron observed, “if we continue to see this trend, it may indicate a more general relationship between polyspecificity and positive charges.”
Antibody Engineering: Online 2023
The upcoming Antibody Engineering: Online event is scheduled for 27 to 28th June 2023. An intensive 2-day meeting, it will delve into the latest in antibody engineering and antibody-based therapeutics, with a focus on AI/ML emerging technologies and antibody optimisation. Download the agenda today to join prominent leaders and scientists as they share new case studies, innovative data, and exciting industry outlooks.
Join Oxford Global’s Antibody Engineering: Online for an exciting opportunity to engage, educate, and elevate. Engage with peers and leading solution providers in an exclusive networking environment. Educate yourself with the latest research and innovation in our renowned conference programme. And elevate your biologics research through immersive, discussion-based sessions within a committed community.
1 https://www.merckgroup.com/en