FAIR Data Principles and Use Cases in Pharma
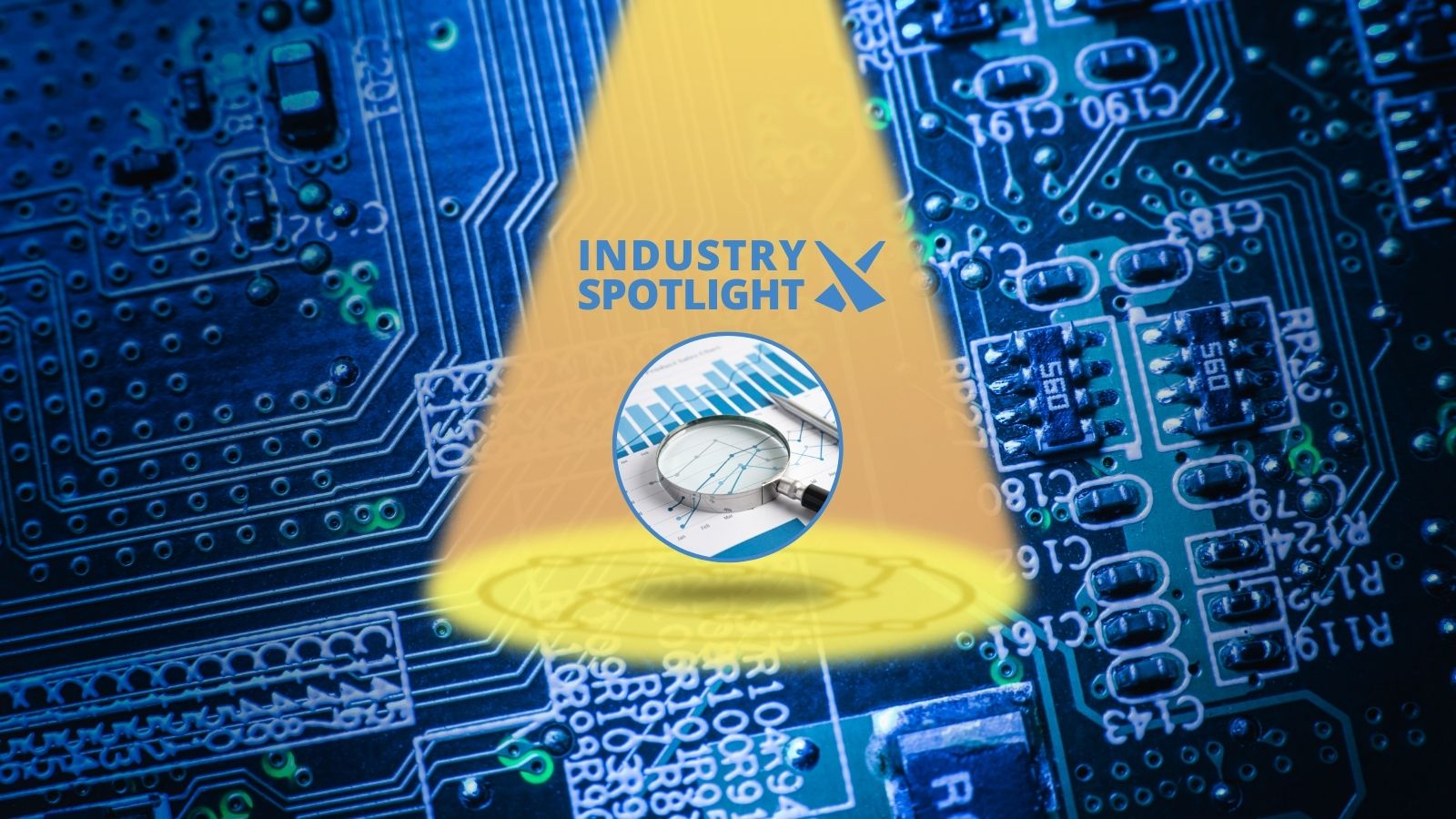
FAIR data principles were first outlined in the journal Scientific Data in 2016 following a consensus between researchers and figures and industry that a higher degree of standardisation was required.
Researchers spend a considerable amount of time, money, and effort collecting data to then interrogate it.
- Understanding trends and risks in clinical data management
- A guide to the successful implementation of real world evidence
- Three major trends transforming how pharma companies use data
A cohesive rollout of FAIR data principles helps to support scientific data management and stewardship, maximising the impact of that investment of time and resources.
As the scientific research and development community has become increasingly globalised, the importance of FAIR data guidelines has only increased, with a growing need for better cultivation and management of the associated digital ecosystem.
FAIR Data Principles
There are four key tenets which constitute the FAIR acronym. They are:
Findability
Data should be assigned globally unique and persistent identifiers to aid in sorting and integrating databases – these tags should clearly and explicitly include the identifier of the data described.
Accessibility
Following on from findability, data should be available under a universally standardised protocol which facilitates an authentication and authorization procedure where necessary.
Interoperability
Metadata should use a formal, accessible, and broadly applicable language for knowledge representation, along with vocabularies that follow FAIR principles and qualified references to other metadata.
Reusability
To facilitate reusability, datasets should be richly described with a variety of accurate and relevant attributes so they can be repurposed appropriately and in concordance with data usage licences.
While there is no expectation that FAIR data principles should be adopted immediately, a sensible approach to implementation would be a gradual introduction for specific use cases and datasets.
FAIR Principles for Data Stewardship
In addition to peer initiatives that focus on data utility for human scholars, FAIR principles place specific emphasis on enhancing the ability of machines to automatically find and use data.
While machines and AI software possess massive benefits in terms of the speed and scale at which they can process data, they lack the contextual understanding possessed by humans.
The FAIRification of data makes it more accessible to humans and machines alike, with process understandings greatly helped by scientific data management.
Proper adherence to the FAIR initiatives should help to facilitate data and knowledge integration, as well as reuse by the community after the data publication process is complete.
However, the existing digital ecosystem surrounding scholarly data publications prevents researchers from extracting the maximum benefit from their work.
FAIR data principles and initiatives can help to push towards achieving these long term initiatives, with improvement of knowledge discovery through assisting humans and their computational agents in access to task-appropriate data.
By implementing FAIR guiding principles for scientific data management, research organisations can massively improve research efficiency and collaborative approaches.
Get your weekly dose of industry news and announcements here, or head over to our PharmaTec homepage to catch up with the latest advances in cellular therapies. To learn more about our upcoming PharmaTec UK conference, visit our event website to download an agenda and register your interest.