Biobank Frameworks: Utilising Federated Machine Learning to Augment Data Solutions
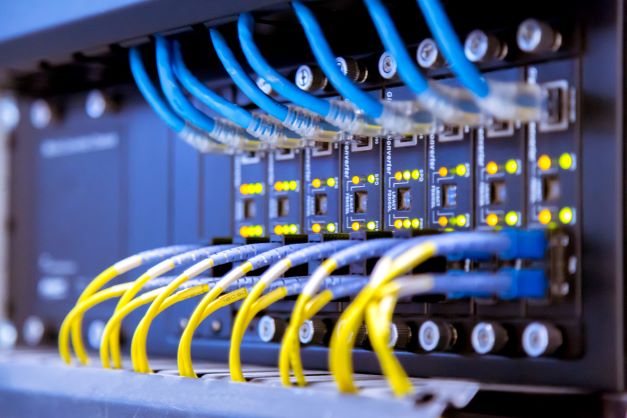
Presented by: Hanati Tuoken, Principal Scientist at Boehringer Ingelheim
Edited by: Ben Norris
The acceleration of data science and its implementation in laboratory environments has given rise to a host of different data infrastructure frameworks. One aspect is a biobank: a collection of human biological samples and associated data, systematically organised for research. For Hanati Tuoken, Principal Scientist at Boehringer Ingelheim, properly implemented biobank frameworks can be an incredibly powerful tool for data management in drug discovery. As he explained at PharmaData UK 2022, recurrent neural networks (RNNs) can incorporate electronic health records (EHRs) and biomarker data to provide a more holistic account of patient data.
Biobank Data in Drug Discovery
A focal impact of the implementation of biobank data is in the acceleration of drug discovery timelines, with the goal of increasing confidence levels for new discoveries. “How do we use patient data from biobanks to run patient stratification?”, asked Tuoken. “What we see in biobanks is they accumulate lots of patient data from different countries.” From these large cohorts, researchers such as Tuoken can find or select patients of interest through criteria selection based on their most relevant diseases.
As Tuoken explained, biobanks offer a first step for locating the most relevant genes to target. “You can have tonnes of cohorts from different countries, and you can cross-validate your findings from different biobanks,” he said. “This is a very unique opportunity that the framework offers.” The mixture of data collected in biobanks is broadly split into genomic and phenotypic data. “There is a huge bank of structured and unstructured text data,” Tuoken added: “tonnes of doctor’s notes, diagnostics, and procedures.”
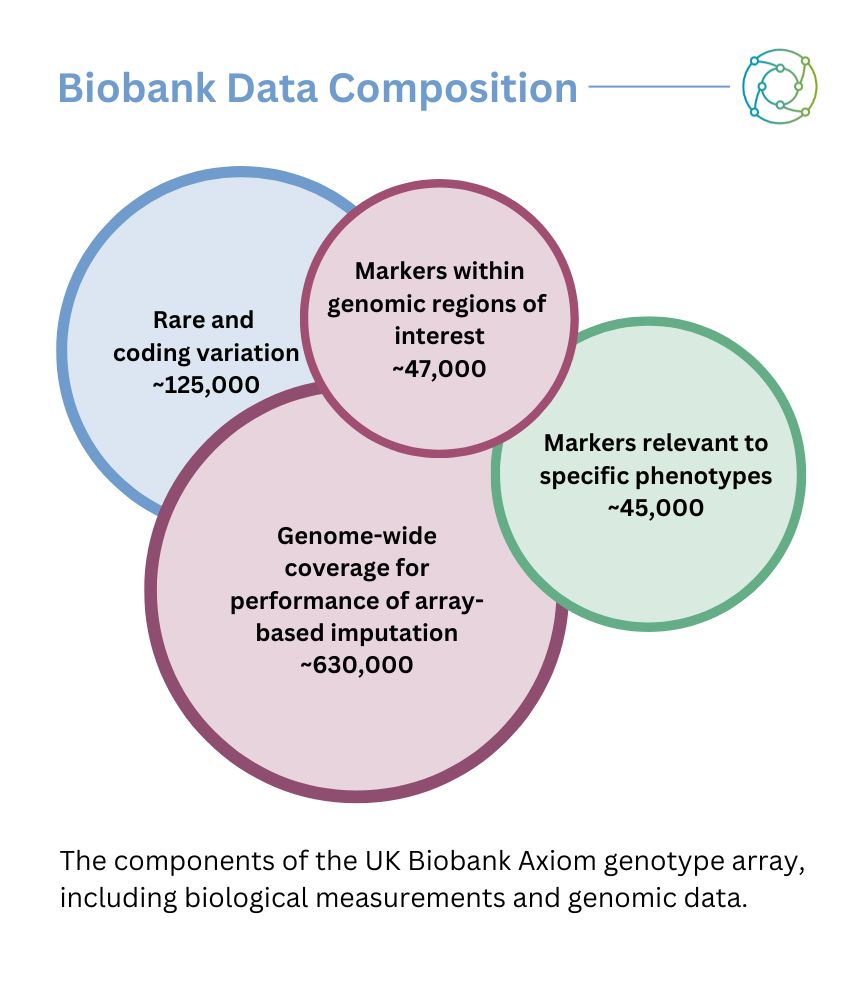
Another data avenue is medical image section data, which may be available in conjunction with biological measurements — such as samples of blood, urine, or other metrics that are measured. “There’s a huge medical image section, which is very popular because it offers another observation besides the doctors’ notes, and you can use it for further analysis,” continued Tuoken. He added that biobank frameworks also featured sequences of genomic data, as well as some transcriptomic sequences.
Examples of Data Collaboration
“I’ve talked about the data,” said Tuoken. “Let’s look into a few examples.” He mentioned the collaboration between the UK Biobank and DNA Nexus, which provides the capabilities for a ‘cleanroom’ containing applications such as cohort browsers. “You can run these knowledge-based deductions,” said Tuoken, “or ask the biobank to transfer your data.” Clinical datasets are relatively conserved within biobank frameworks: the issue of different access platforms also presents challenges when integrating data.
Across different organisations and collectives, each biobank has its own platform. “Different biobanks have different accessibility, but one thing they all have in common is they all have clinical data,” said Tuoken. With the cohort, use cases can be considered for patient stratification. “Generally, let’s say you have a cohort and you have some inclusion and exclusion criteria — the approach is okay for some diseases, but for some undiagnosed diseases it’s hard to select the treatment cohort.”
- Real-World Data in Real-World Solutions: Future Approaches to Healthcare Provision
- Moving Towards End-to-End Automation Through Heightened Lab Efficiency
- Telerobotics in Healthcare: From Space Robotics to the Mobile Lab Robotics of the Future
By syndicating with biobank data, researchers can increase the size of the treatment control group, providing them with more power in conducting follow-up downstream analysis. One example of this could be the use of known biomarkers as a sign of normal or abnormal processes: a limitation of this approach is that disable treatment groups can be hard to define for under-diagnosed diseases using current approaches.
Biobank Frameworks and Recurrent Neural Networks
One approach to stratifying patient data is the use of deep learning approaches through the implementation of recurrent neural networks. Tuoken gave the example of clinical data from prescriptions, procedures, diagnoses, and medical notes. “All of this data can be used as an input for a recurring network — we can treat it as a natural language to figure out if there’s any hidden message in this simple binary decision.” By analysing data sets in this way, deep learning approaches could detect trends in particular patient groups which may be related to their condition.
RNNs trained with EHRs and biomarkers can improve the precision of patient detection for those with rare diseases. “We just take a sequence of diagnoses, and we let it go through an embedding layer and feed it to the RNN,” explained Tuoken. “Then we concatenate it back on the output — if it’s a predictive disease, we can predict a simple yes or no.” He added that by analysing the data in this way, Boehringer Ingelheim had observed an increase in precision using the RNN compared with the inclusion and exclusion approach.
“We want to build on this further,” Tuoken continued, “and the reason we want to do this is we want to replicate this in biobanks.” The integration of EHRs from multiple biobanks using federated learning networks. “We are ensuring this sensitive information and regulation is protected, and we are ensuring all of this biobank data is relevant data,” added Tuoken. “We are just trying to figure out which is the best way to do this.”
Federated Machine Learning in Biobank Frameworks
“I think federated machine learning is becoming more and more popular,” said Tuoken as he rounded off his presentation. “Medical imaging is easy to understand — assuming that you have lots of images from different biobanks, you can run this federated machine learning, and for these image models I think it’s even more easy to aggregate the weight.” He added that Covid-19 was one good example for federated machine learning, and said he felt the method would offer a strong basic approach for decentralised data.
“I think federated learning is becoming more and more popular… and I think we will see it accelerate drug discoveries.”
“Whether we do it federated or not, data quality is always a big topic,” added Tuoken. “The focus has to be on the data quality — we don’t want to interpret too much.” Data quality, bias, and standardisation are equally important to account for when planning for federated and non-federated learning factoring in recurrent neural networks. “I think federated learning will offer us a lot of opportunities in the future, and I think we will see it accelerate drug discoveries,” Tuoken concluded.
Want to read more about the latest advances in PharmaData? Head over to our PharmaTec portal to get insights from the industries best and brightest. If you’d like to register your interest in our upcoming Mobile Robotics: In-Person event, click here.