Accelerating Target Deconvolution for Therapeutic Antibody Candidates
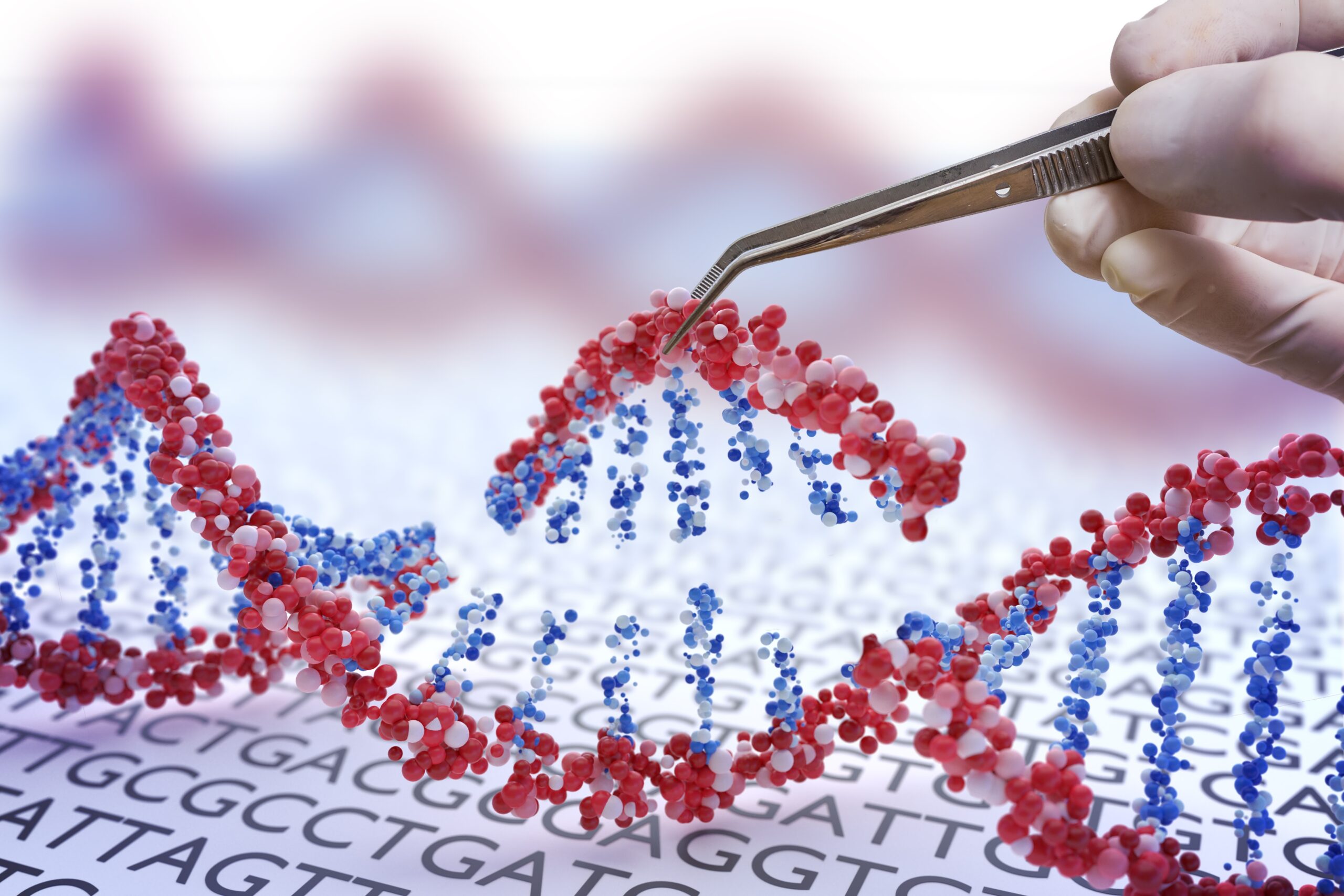
Presented by: Jenny Mattsson, Senior Research Engineer at BioInvent International AB
Edited by: Cara Digby-Patel
Phenotypic discovery enables the identification of therapeutic antibodies towards novel targets. These therapeutic antibodies, which can target tumours directly or the tumour microenvironment, have transformed cancer patient survival rates. However, many patients either will not respond to the drugs or develop resistance. Therefore, there is a need for new targets and drugs to be identified.
This is where BioInvent’s work comes in. Speaking at Oxford Global's Discovery Europe: In-Person conference, Senior Research Engineer Jenny Mattsson discussed how BioInvent utilises highly parallelised genome editing for antibody target deconvolution. This process is quicker and has a far higher success rate than other commonly used approaches in this field.
The BioInvent Pipeline
Mattsson first gave an overview of BioInvent’s phenotypic discovery pipeline, which uses a target-agnostic functional approach to identify new antibodies and targets. First, the desired cell type is selected; these can be either cancer cells or important effector cells, such as regulatory T cells or tumour-associated myeloid cells (TAM).
Having identified and isolated the target cells of interest, the company’s researchers perform a screening using a recombinant phage display library. This allows them to find antibody fragments that can target structures which are upregulated on the cells of interest. The antibodies are tested as full-length IgGs in functional assays, but their molecular target is still unknown. When antibodies with interesting functional profiles are identified, they are included in a target deconvolution effort.
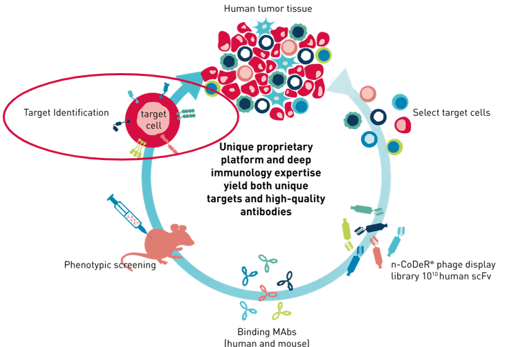
Mattsson’s presentation focused on target deconvolution which is “widely recognised as a bottleneck in the phenotypic discovery process”. Commonly used techniques include immunoprecipitation followed by mass spectrometry or overexpression libraries. However, BioInvent has established a new approach which uses CRISPR/Cas9 knockout screening to identify targets. Mattsson explained this method with reference to a successful study to illustrate the efficacy of BioInvent's technique.
Target Deconvolution Using CRISPR/Cas9 Screening
In the case study presented, researchers identified target cells that the antibodies of interest recognise. These cells could either be a primary cell or a cell line, but they had to be transducible.
Antibodies which target regulatory T cells, TAM cells, and cancer cells were selected. However, these cells are not readily available and are not particularly suitable for genome-wide CRISPR screening, so BioInvent tested the binding of these antibodies to other cell types.
- Examining Target Identification and Validation in Drug Discovery
- Shooting for the StaRs: Stabilising GPCRs and Mapping Their Structure
- AlphaFold: Momentary or Revolutionary?
The antibodies were found to bind to a range of cell types, including Jurkat, H9 and THP-1 cell lines, and primary CD4+ cells. Researchers also included two control antibodies which target the CD2 and CD45 antigens, bringing the number of antibodies to 39.
The cells were then transduced with genome-wide CRISPR knockout libraries so that each cell was infected with a single lentivirus particle carrying the genes for one sgRNA. This resulted in a heterogeneous cell population where most cells were still positive for the target antigen, and a few were antigen-negative.
Researchers then stained the antibodies of interest and sorted the most fluorescent negative cells. The most fluorescent positive cells were also sorted as controls. This produced a cell population with an enrichment of antigen-negative cells compared to the unsorted cells. With cells lines, the cells were expanded and a second sort performed, which resulted in an even more pronounced enrichment of antigen-negative cells.
DNA was extracted from the sorted and the control cells, and Illumina NextSeq sequencing was performed to identify sgRNA in these populations. BioInvent conducted MAGeCK analyses using a robust ranking aggregation algorithm to identify genes with enriched mRNA in negative cells compared to positive. Mattsson explained that for each of the 39 antibodies examined, BioInvent sorted three to five replicates depending on whether a second sort was completed. The final MAGeCK scores came from a combined analysis of all replicates.
Target Gene Prioritisation
BioInvent prioritised target genes using three different criteria. Firstly, by identifying cell membrane genes with significant MAGeCK scores (a false discovery rate of less than 5%). A single gene was identified for twenty-four antibodies, whilst two related genes were identified for nine antibodies.
The second criterion was whether the antibodies had an enriched MHC class I dependency gene expression. A set of 11 dependency genes that are important for MHC class I expression was defined, including genes which encode surface heterodimer, peptide loading complexes, and transcriptional regulator genes. Overall, 13 antibodies with significant MHC class I enrichment scores were identified. This left four antibodies which did not have a prioritised target. Therefore, the third criterion established was the highest-ranked nonsignificant cell membrane gene.
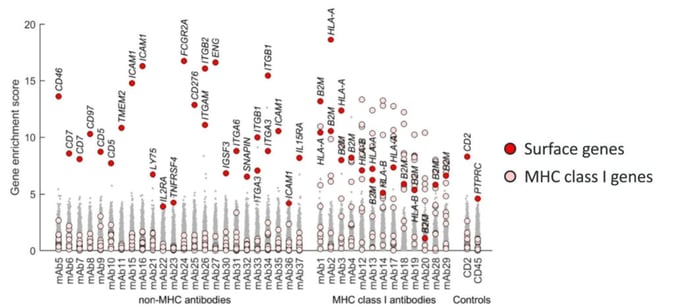
Target Validation
Researchers used three approaches for target validation, which follows target identification. The first was an enzyme-linked immunoabsorbent assay (ELISA) which coated the extracellular domain of the proposed target in plates and then detected using the test antibodies. This technique validated the specificities of twenty antibodies.
Block assay was the second approach used. Antibody binding to the proposed target was tested with and without a block, in this case a polyclonal antibody. Without the block, the selected antibody will bind, but with the block, if the polyclonal antibody has the same target, binding is significantly reduced or absent (see Figure 3). BioInvent were able to verify nineteen targets using this method.
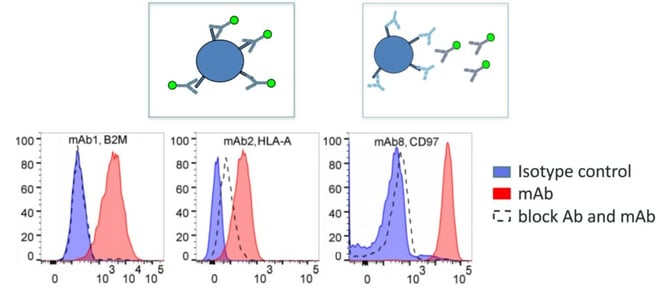
The third approach was overexpression. This technique begins with a cell which is initially negative for the target antigen. Then, after overexpression or transfection with the proposed target gene, the cell will become positive if the proposed target is correct. This process allowed researchers to validate the specificity of three antibodies. Using these three approaches, researchers validated the specificity of 34 out of the 39 antibodies.
BioInvent's findings have immediate implications for the implementation of phenotypic discovery, as they allow researchers to overcome the bottleneck which target deconvolution has previously presented.
Four of the outstanding antibodies were proposed MHC class I binders. Due to the variability of targets with MHC class I binding, it can be used to predict and validate targets. Therefore, researchers compared the MHC class I enrichment scores of these four antibodies to those with validated MHC class I activity. They found that the enrichment scores were very similar, allowing them to infer that the four unvalidated antibodies are MHC class I specific. This brought the total number of deconvoluted targets to 38 out of 39.
Conclusion
Mattsson closed by touching on the fact that CRISPR screens also allowed researchers to find target-related biology via dependencies for various factors. Mattsson had already touched on this when discussing how MHC class I dependency can be used during target gene prioritisation. Other factors that allow target-related biology to be established based on dependencies include integrin dependency and transcription factor dependencies.
Overall, these data established CRISPR/Cas9 as an effective target deconvolution approach, with a higher success rate than existing approaches. BioInvent's findings have immediate implications for the implementation of phenotypic discovery, as they allow researchers to overcome the bottleneck which target deconvolution has previously presented.
For the original peer-reviewed and published research article, please see: Mattsson, J., Ekdahl, L., Junghus, F. et al. Accelerating target deconvolution for therapeutic antibody candidates using highly parallelized genome editing. Nat Commun 12, 1277 (2021). DOI: https://doi.org/10.1038/s41467-021-21518-4
Join and network with leaders in drug discovery at Organ Modelling UK, where we will address methods to optimise organ modelling techniques and facilitate advanced applications.